Unveiling Bias in Medical AI Models
July 2024
MIT News
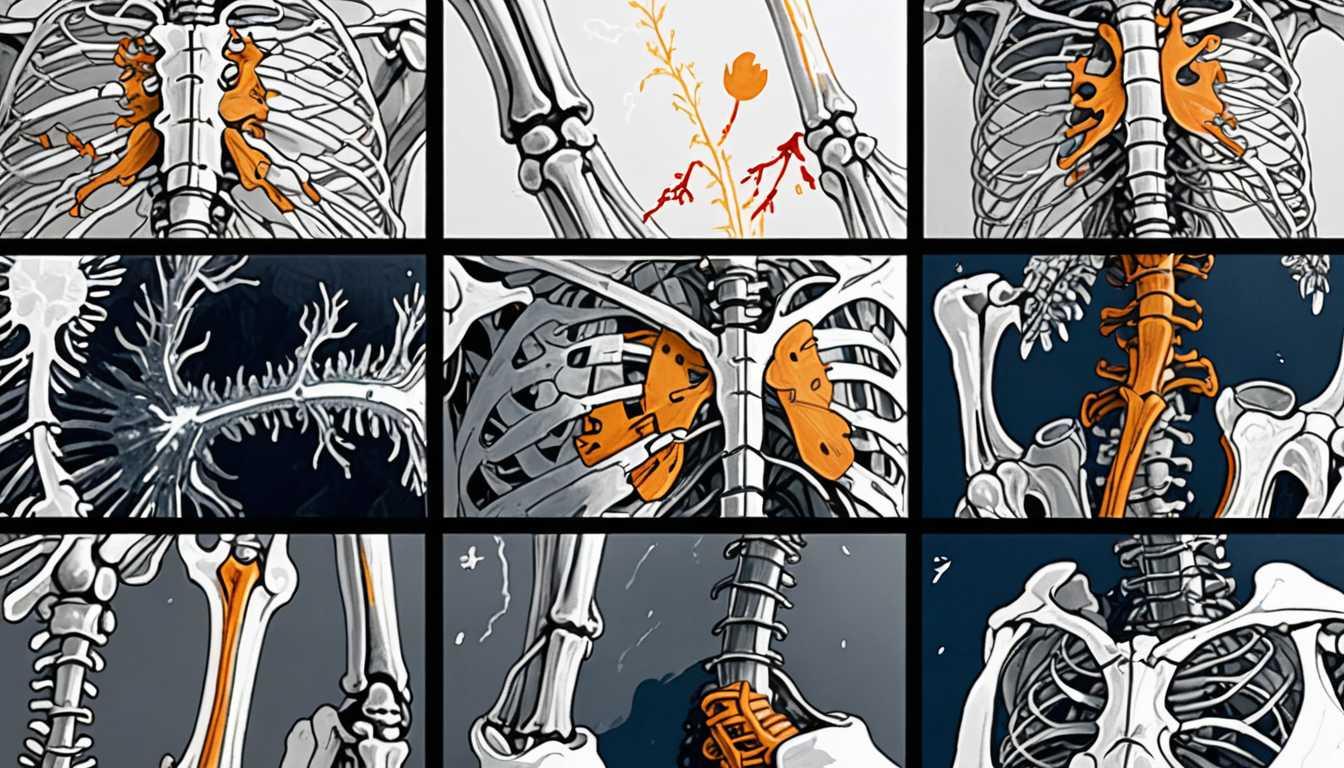
Introduction
Dive into the fascinating world of AI in medicine with MIT's groundbreaking study on bias in medical image analysis models. Discover how these models predict patient demographics from X-rays and learn how researchers are striving to make them fairer for all groups.
READ FULL ARTICLEWhy It Matters
Discover how this topic shapes your world and future
Unveiling the Biases in AI Medical Diagnoses
Artificial intelligence (AI) plays a crucial role in medical diagnoses, particularly in analyzing images like X-rays. Recent studies have revealed that AI models may not perform equally across different demographic groups, showing disparities in accuracy, especially among women and people of color. Surprisingly, these models have demonstrated the ability to predict a patient's race accurately from chest X-rays, surpassing even skilled radiologists. However, researchers have found that these models exhibit "fairness gaps," leading to inaccuracies in diagnosing individuals from various racial and gender groups. This highlights the significance of understanding and addressing biases in AI algorithms used in healthcare settings, ensuring fair and accurate medical assessments for all individuals.
Speak like a Scholar

Demographic shortcuts
Simplified methods used by AI models to make diagnostic evaluations based on demographic attributes, potentially leading to biased outcomes.

Fairness gaps
Discrepancies in the performance of AI models in accurately diagnosing individuals from different demographic groups.

Debiasing
Techniques aimed at reducing biases in AI models, enhancing their fairness and accuracy in medical diagnoses.

Subgroup robustness
Training models to improve performance on demographic subgroups with the worst accuracy.

Group adversarial approaches
Strategies to remove demographic information from images, promoting unbiased predictions by AI models.

Off•the•shelf model
A pre•built AI model that can be readily deployed in various healthcare settings without extensive customization.
Independent Research Ideas

Investigate the Impact of Demographic Bias on AI Medical Diagnoses
Explore how demographic shortcuts influence the accuracy of AI models in diagnosing medical conditions across different population groups.

Ethical Considerations in AI Healthcare Algorithms
Analyze the ethical implications of using AI in medical diagnoses and the importance of ensuring fairness and accuracy.

Interdisciplinary Study of AI and Healthcare Disparities
Examine the intersection of AI technology and healthcare disparities, focusing on strategies to mitigate biases in medical AI models.

Comparative Analysis of Debiasing Techniques in AI
Compare the effectiveness of subgroup robustness and group adversarial approaches in reducing biases in AI algorithms for medical imaging.

Future of AI in Healthcare
Predict the advancements and challenges of integrating AI technology into healthcare systems, considering the implications for patient care and equity.
Related Articles
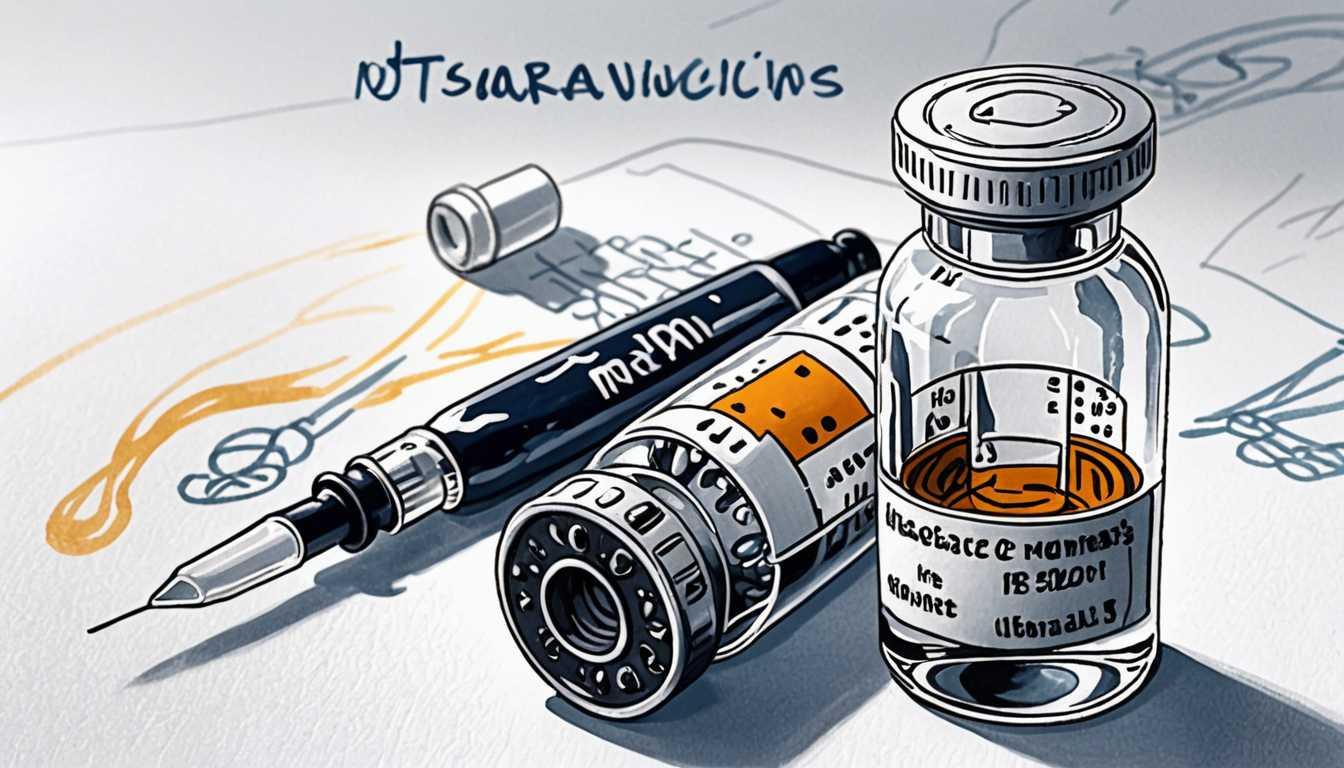
Outsmarting Pandemics: A New Vaccine Play
June 2023
Cornell University
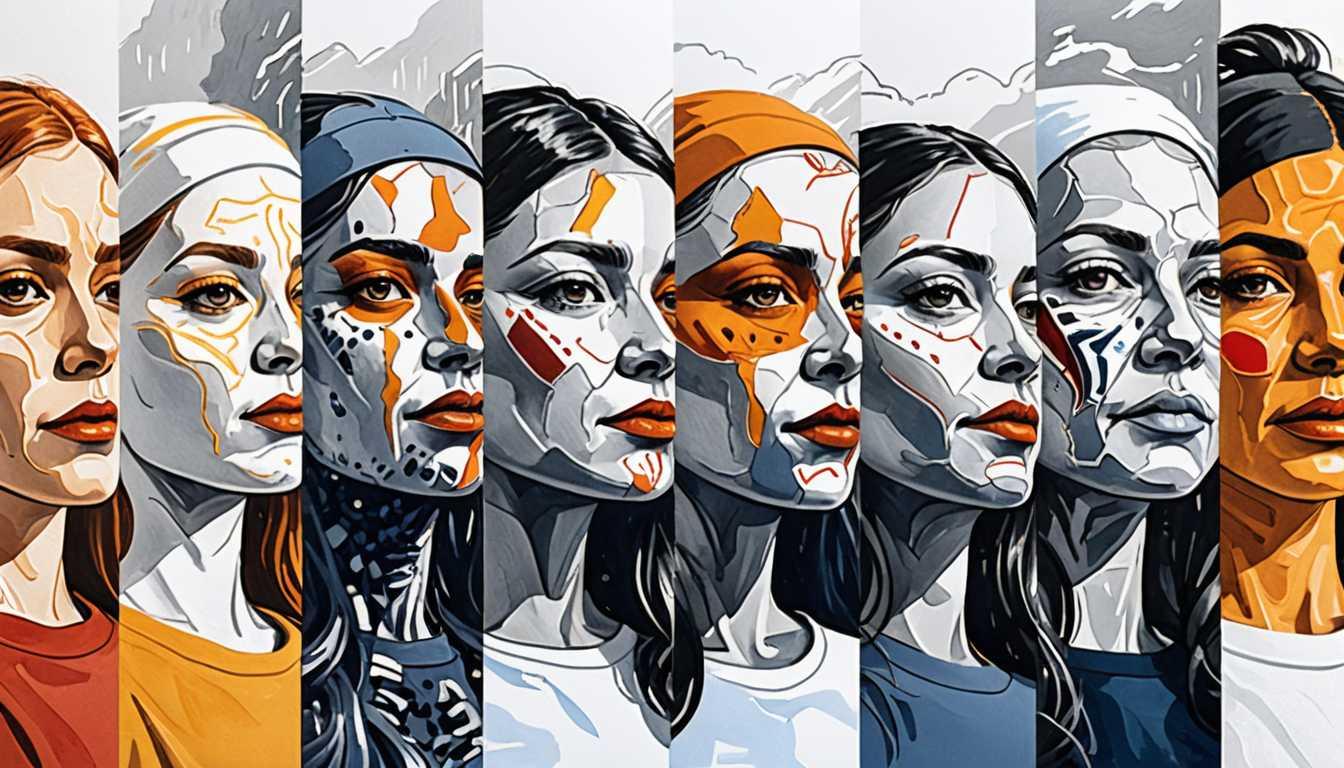
AI Bias in Healthcare Unveiled
August 2023
Massachusetts Institute of Technology (MIT)
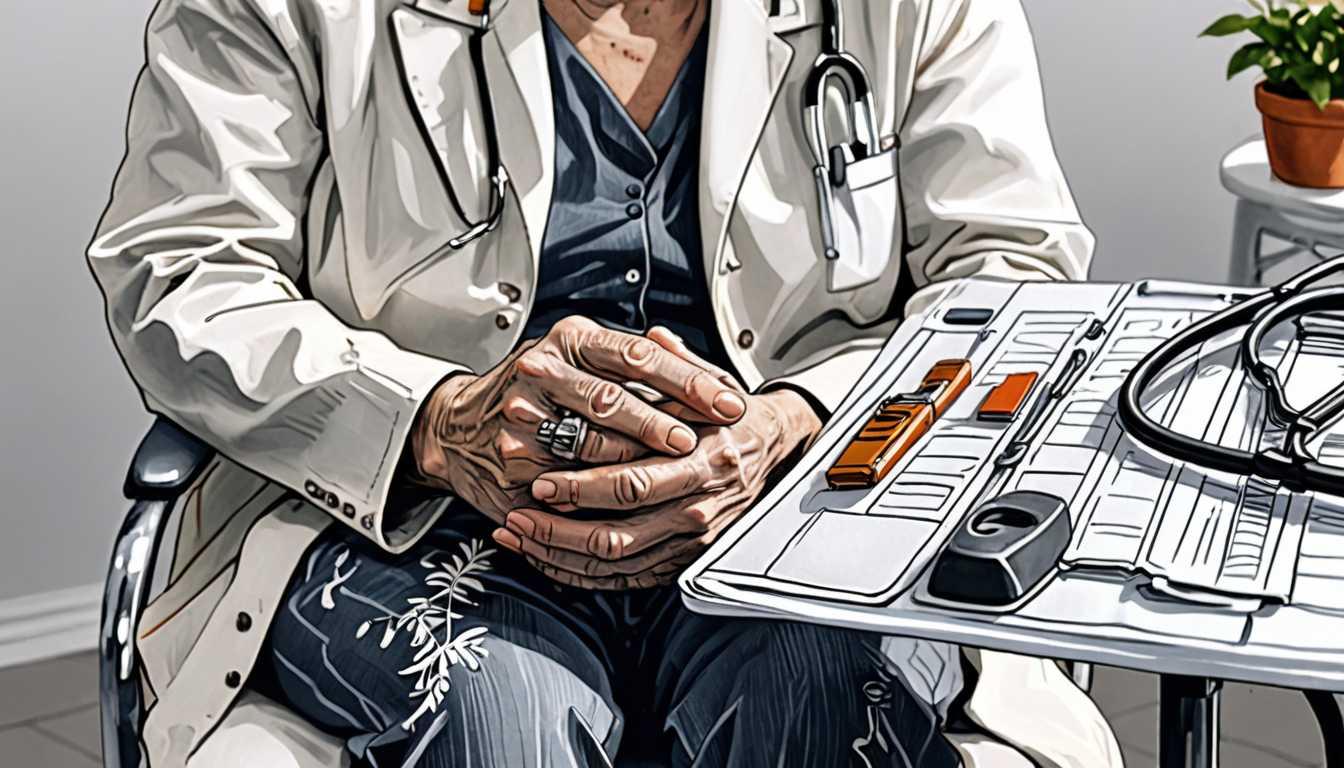
AI: A Game Changer in Patient Care!
May 2024
Cornell News Highlights
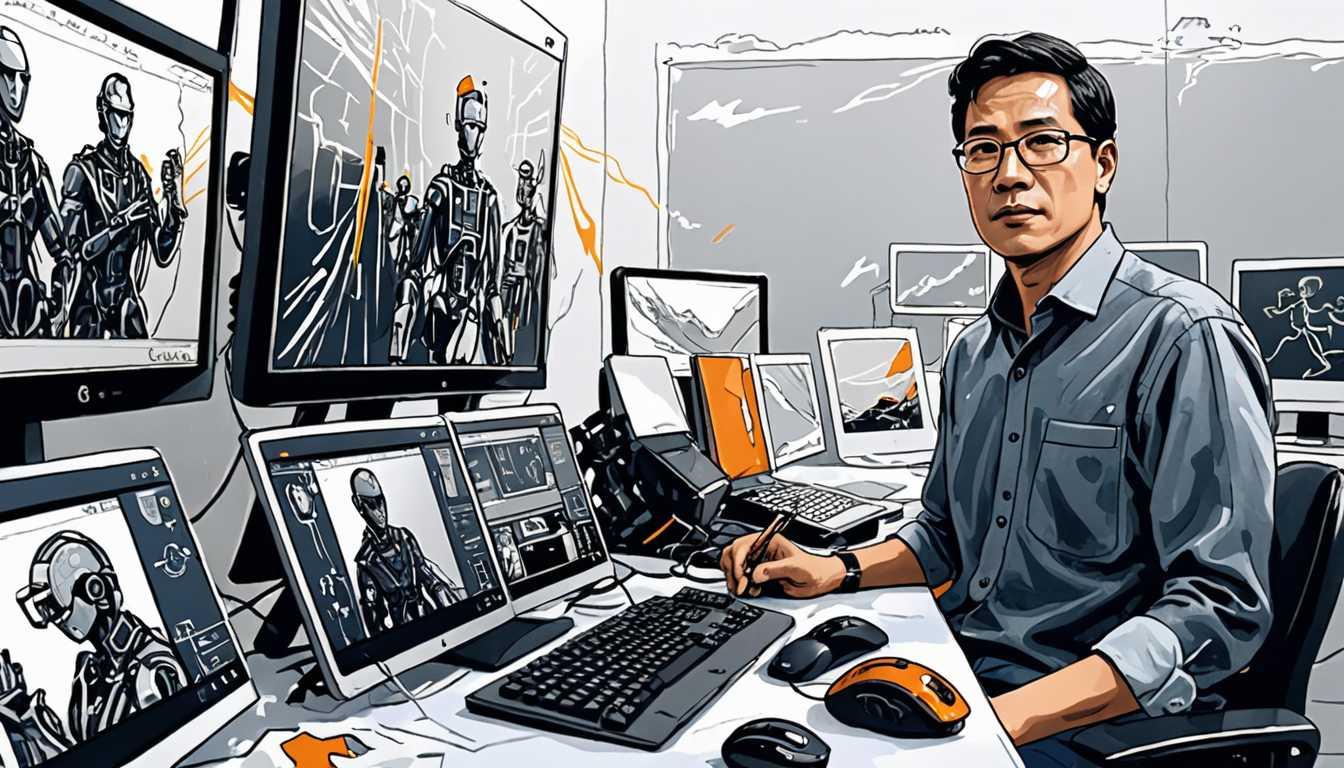
AI: The Future of Faster Cancer Care
June 2023
University of Cambridge
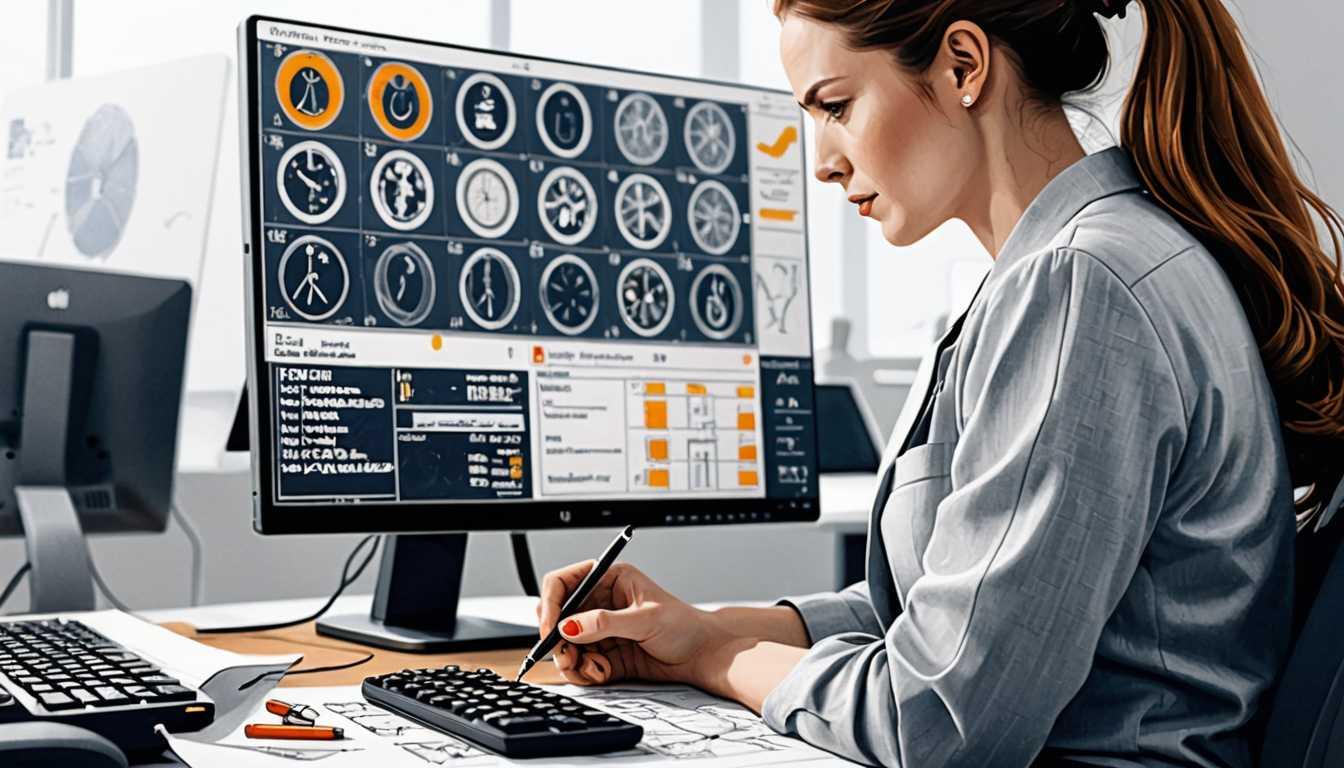
AI: Healthcare's Double-Edged Sword
August 2023
Stanford University