AI Bias in Healthcare Unveiled
August 2023
Massachusetts Institute of Technology (MIT)
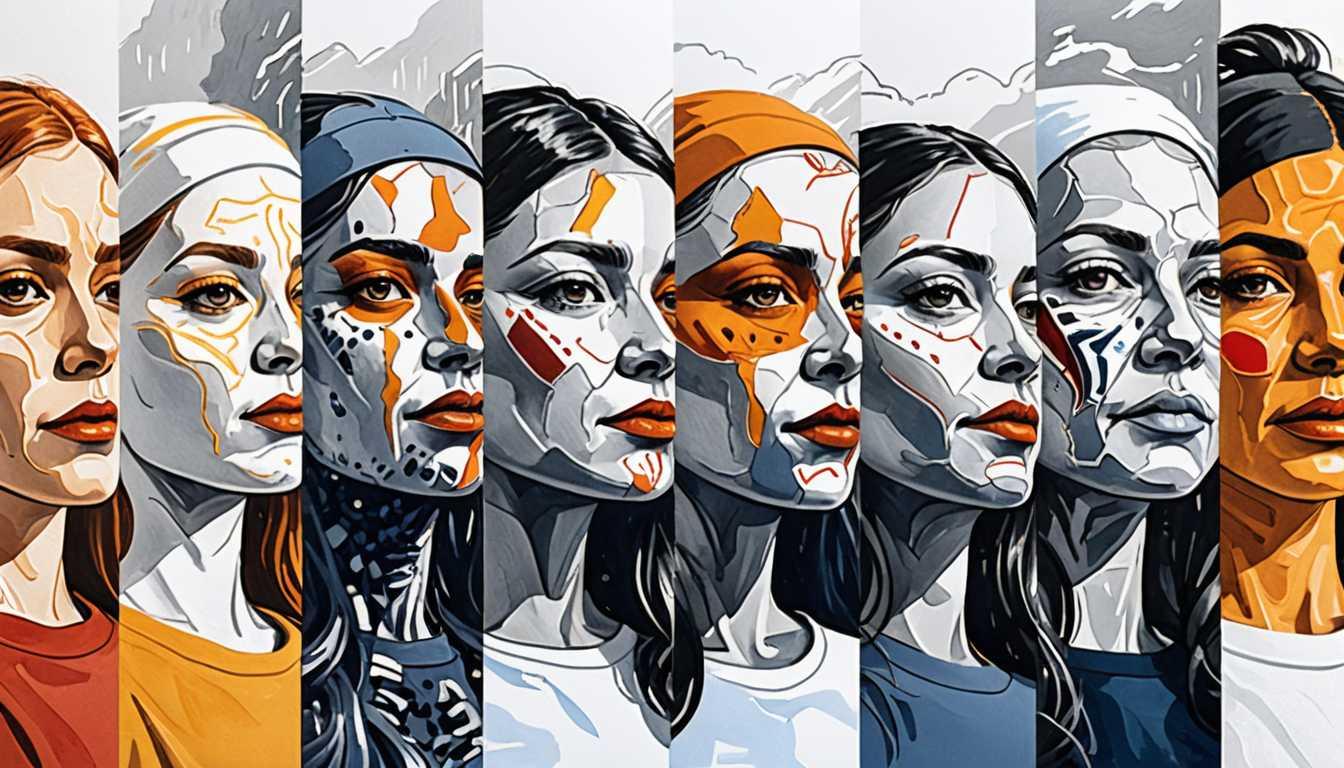
Introduction
Dive into the fascinating world of AI and healthcare with an article from MIT! Discover how Marzyeh Ghassemi and her team are uncovering biases in machine learning that could skew medical diagnoses and treatments. From cows on grass to gender disparities in pneumonia detection, this study sheds light on the urgent need for more equitable AI models. Ready to explore the cutting-edge intersection of technology and medicine? This article is your gateway!
READ FULL ARTICLEWhy It Matters
Discover how this topic shapes your world and future
Unveiling the Bias Behind the Screen
Imagine going to the doctor and getting a diagnosis that’s not quite right, simply because the computer program the doctor used didn’t have enough data from people like you. This scenario isn’t from a sci-fi movie; it’s a real issue in the world of machine learning in healthcare. Researchers like Marzyeh Ghassemi are on a quest to understand why and how these biases happen. Their work is crucial because it impacts everyone's health and well-being globally. By figuring out where biases in machine learning come from, we can work towards medical diagnoses and treatments that are fair and accurate for everyone, regardless of who they are or where they come from. This topic might seem a bit out there, but it’s about making sure everyone gets the same shot at getting better when they’re sick, which is something that could affect you or someone you care about someday.
Speak like a Scholar

Subpopulation shifts
Variations in how well a machine learning model performs for different groups of people, often leading to biases.

Spurious correlations
Incorrect connections made by a model between two things because it doesn’t understand the context (like assuming cows can only be on grass).

Attribute imbalance
When the data used to train a machine learning model is unevenly distributed across different characteristics (like more men than women).

Class imbalance
A disparity in the number of examples across different categories in training data, such as having many more healthy subjects than sick ones.

Attribute generalization
The ability of a model to make accurate predictions about a group even when it has limited data about that group.

Encoder and classifier
Parts of a neural network where the encoder processes the input data and the classifier makes the final decision or prediction.
Independent Research Ideas

Investigate the impact of diverse data in training machine learning models
Explore how including a wide range of data from different populations can improve the accuracy and fairness of medical diagnostics.

The role of cultural competence in machine learning
Examine how understanding and integrating cultural differences into machine learning models can reduce biases in healthcare.

Ethical implications of AI in healthcare
Delve into the ethical considerations of using AI in medical diagnosis and treatment, focusing on privacy, consent, and the right to fair treatment.

Machine learning in emergency medicine
Investigate how biases in machine learning models could affect decision-making in high-stakes environments like emergency rooms.

The future of personalized medicine through AI
Explore the potential for machine learning to offer personalized medical treatment plans and how to ensure these models are equitable for all patients.
Related Articles
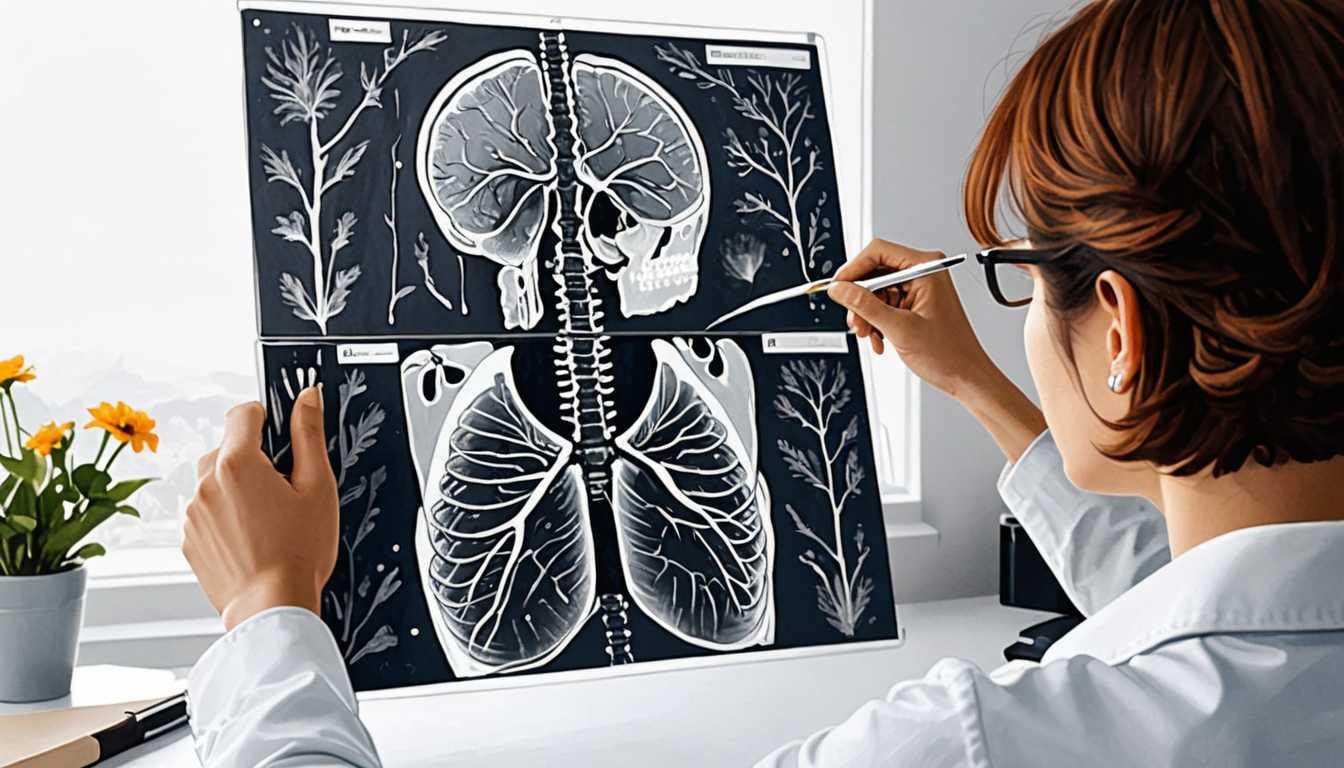
Mia: AI's Triumph in Cancer Detection
November 2023
Imperial College London
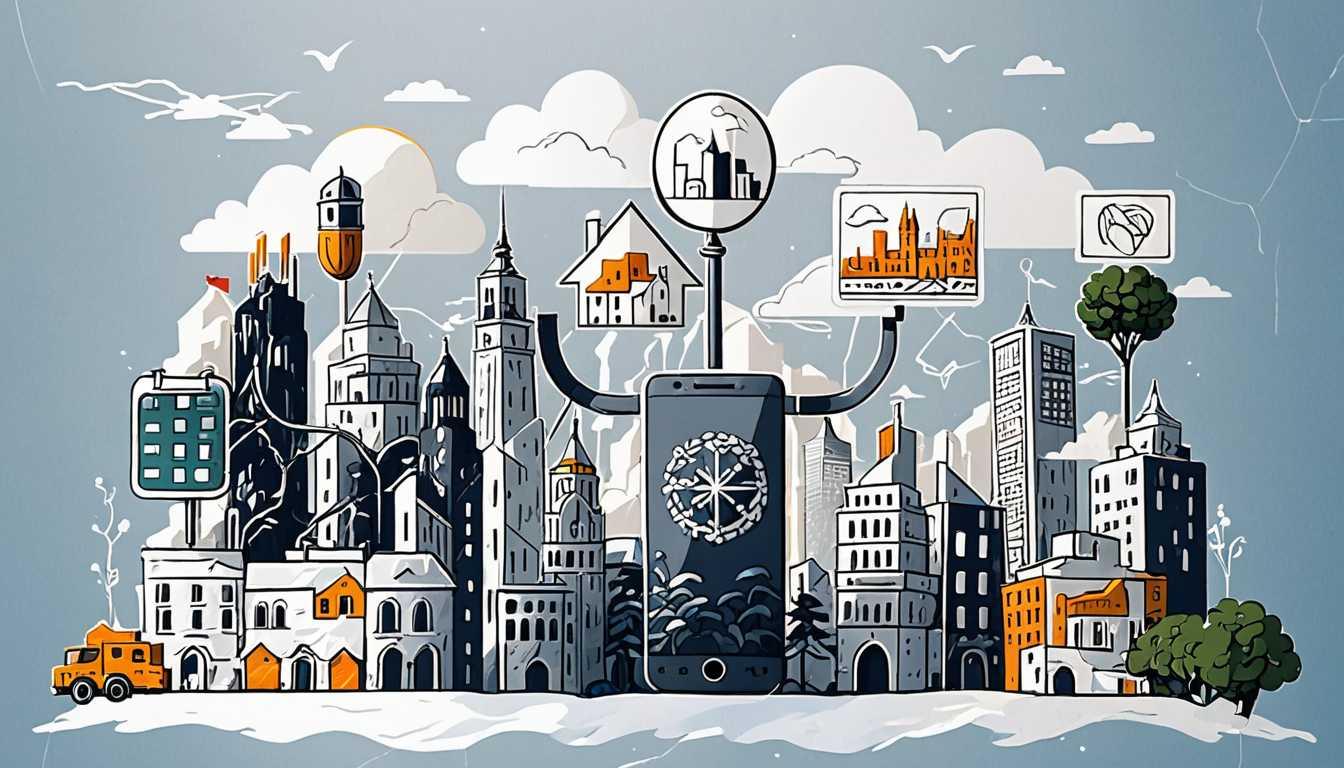
AI: The Future of Medicine
July 2023
MIT Technology Review
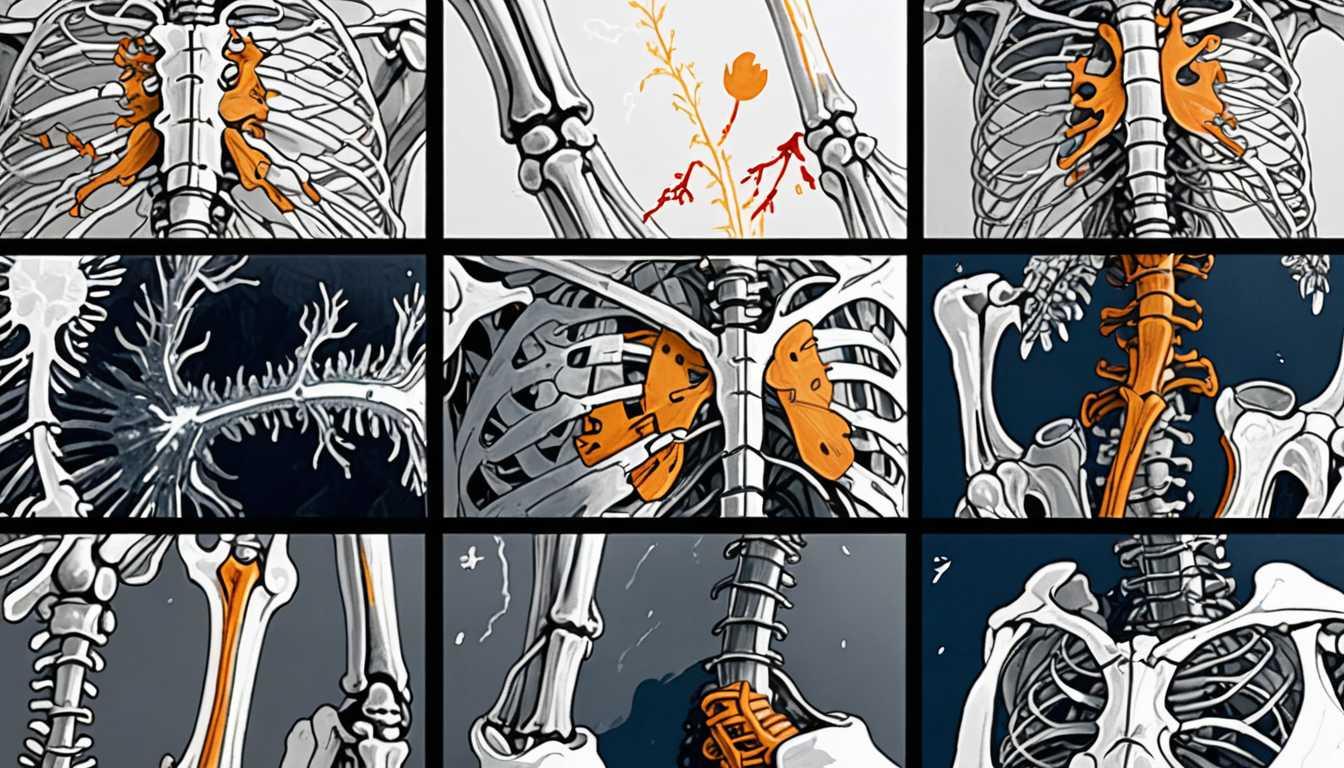
Unveiling Bias in Medical AI Models
July 2024
MIT News
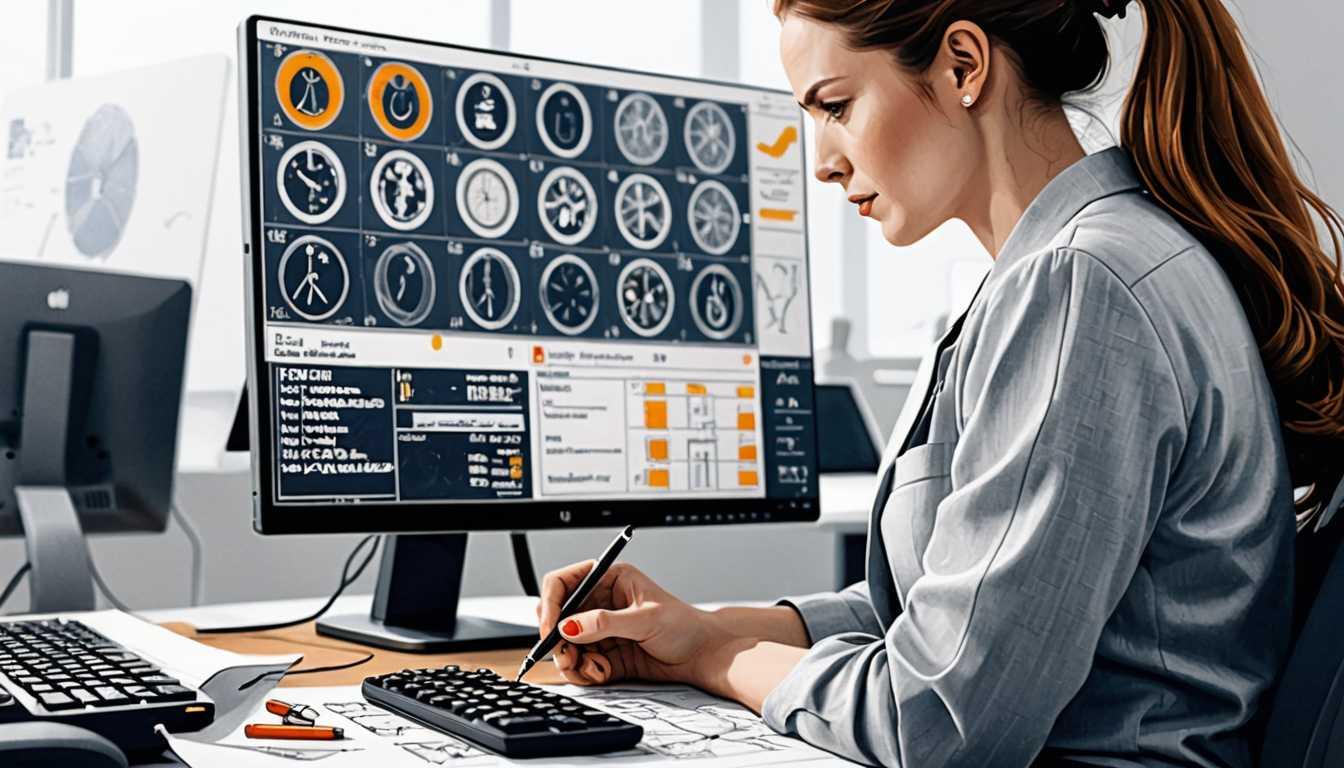
AI: Healthcare's Double-Edged Sword
August 2023
Stanford University
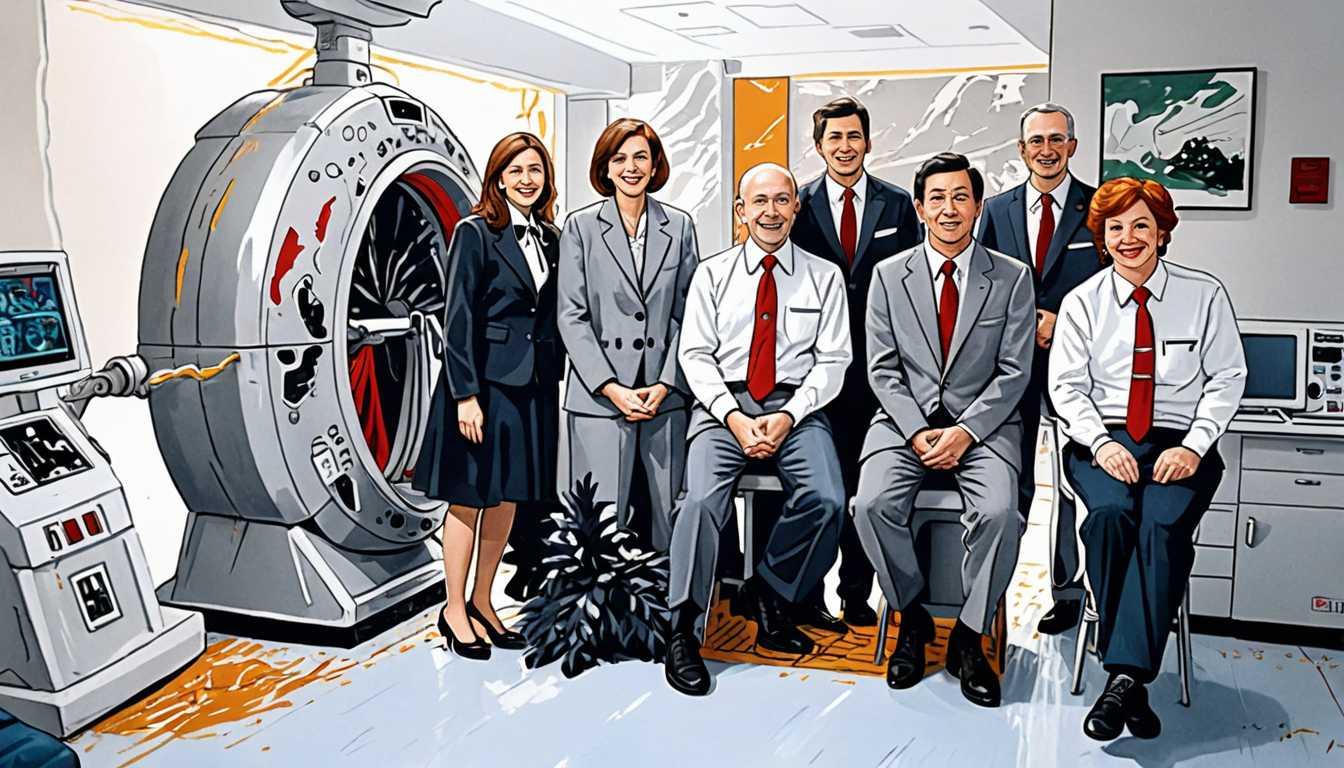
Sybil: AI's Leap Against Lung Cancer
January 2023
Massachusetts Institute of Technology (MIT)