AI Trust: MIT's Uncertainty Breakthrough
July 2024
MIT News
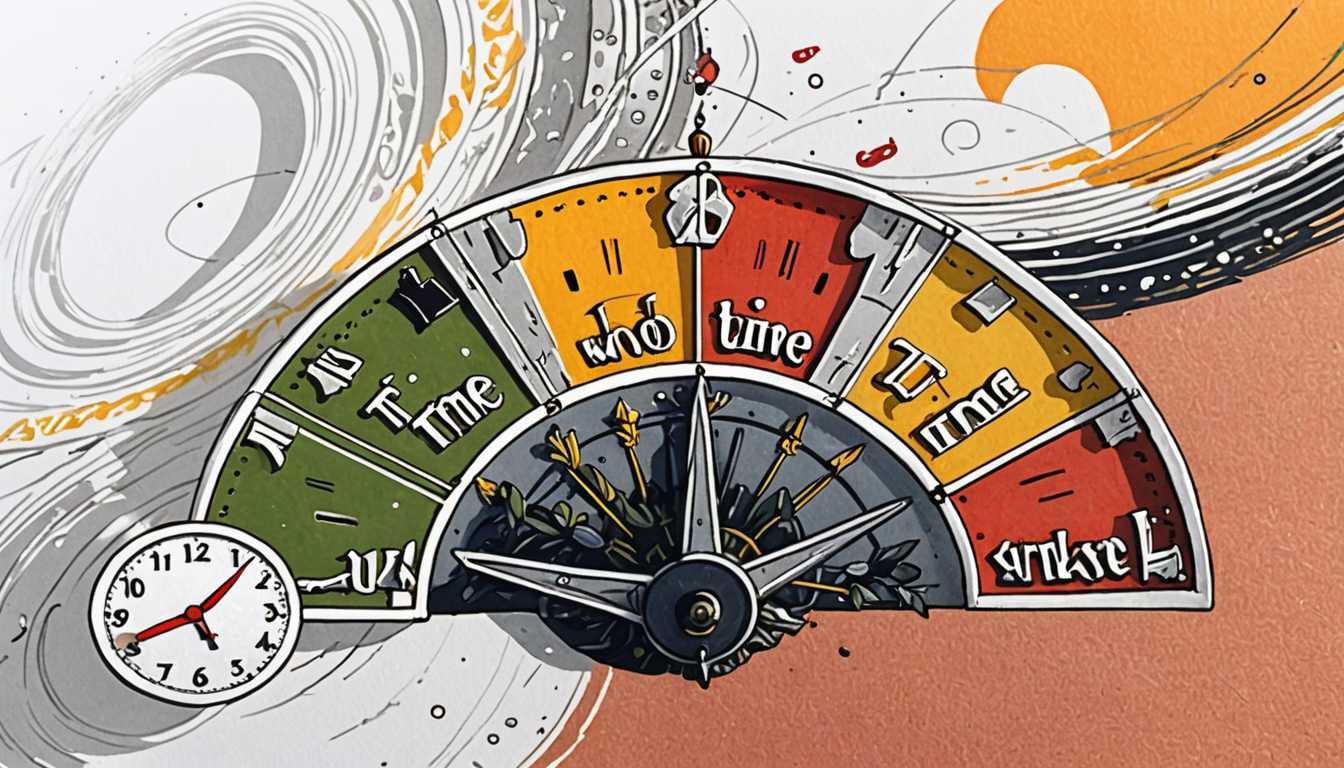
Introduction
Ever wondered when to trust AI models? MIT researchers have cracked the code, improving uncertainty estimates efficiently for crucial tasks like medical image analysis and job application filtering. Dive into this cutting-edge technique that could revolutionize how we interact with machine-learning models.
READ FULL ARTICLEWhy It Matters
Discover how this topic shapes your world and future
Navigating the Uncertainty of Machine Learning
Understanding how machine learning models work is becoming increasingly important in today's data-driven world. These models are used in various critical fields, such as healthcare, hiring processes, and even self-driving cars. However, an essential aspect that researchers are focusing on is the concept of uncertainty. When a machine-learning model makes a prediction, it should also communicate how confident it is about that prediction. For example, if a model identifies a health issue in a medical image, knowing its level of confidence can help doctors make better decisions. Recent advancements, like the new method from MIT researchers, improve how accurately these models express their uncertainty, which is vital for ensuring safety and reliability. This relates directly to you, as understanding these concepts can impact your future, especially if you're interested in technology, healthcare, or data science.
Speak like a Scholar

Machine Learning
A type of artificial intelligence where computers learn from data to make predictions or decisions without being explicitly programmed for each task.

Uncertainty Quantification
The process of determining how confident a model is about its predictions, which is crucial for making informed decisions.

Calibration
Adjusting a model’s predictions so they accurately reflect its true confidence levels, ensuring that the predictions are reliable.

Stochastic Data Complexity
A measure of how much information is needed to describe a data point, less complexity indicates higher confidence in the model's prediction.

Influence Function
A mathematical function used to assess how changes in data can affect a model's predictions, helping to understand and improve the model's performance.

Counterfactual Information
Hypothetical scenarios that challenge the model's original predictions, allowing researchers to test how confident the model really is about its choices.
Independent Research Ideas

The Role of Machine Learning in Disease Diagnosis
Investigate how machine learning is transforming medical diagnostics and the implications of its accuracy on patient care.

Comparative Analysis of Uncertainty Quantification Methods
Explore different techniques for quantifying uncertainty in machine learning and their effectiveness in various applications.

Ethics of Machine Learning in Hiring Practices
Examine the ethical implications of using machine learning models in recruitment and how uncertainty affects fairness in hiring.

Impact of Uncertainty on Self-Driving Technology
Research the challenges and advancements in machine learning regarding the uncertainties faced by autonomous vehicles.

Applications of Minimum Description Length in Other Fields
Investigate how the principle of minimum description length can be applied beyond machine learning, perhaps in fields like natural language processing or environmental science.
Related Articles
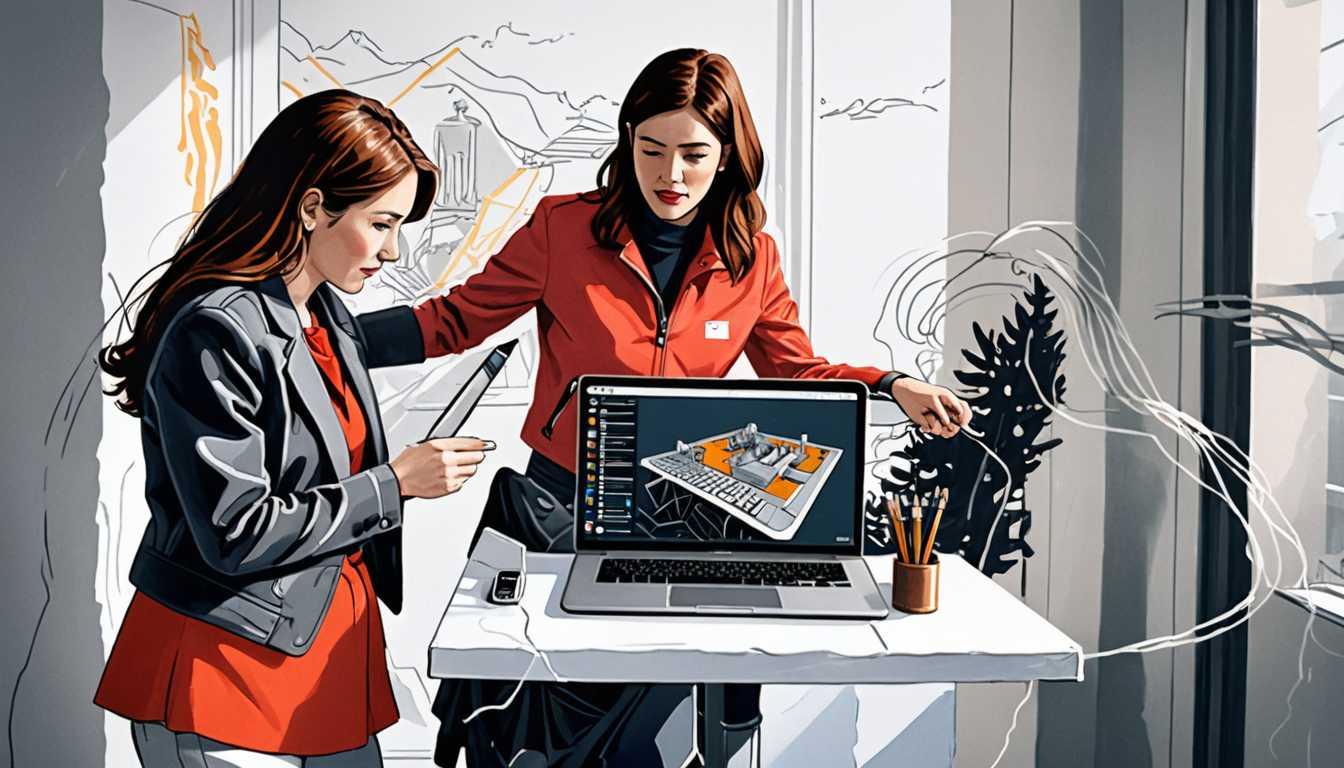
iPhones Unlock Biomechanics Secrets
October 2023
Stanford University
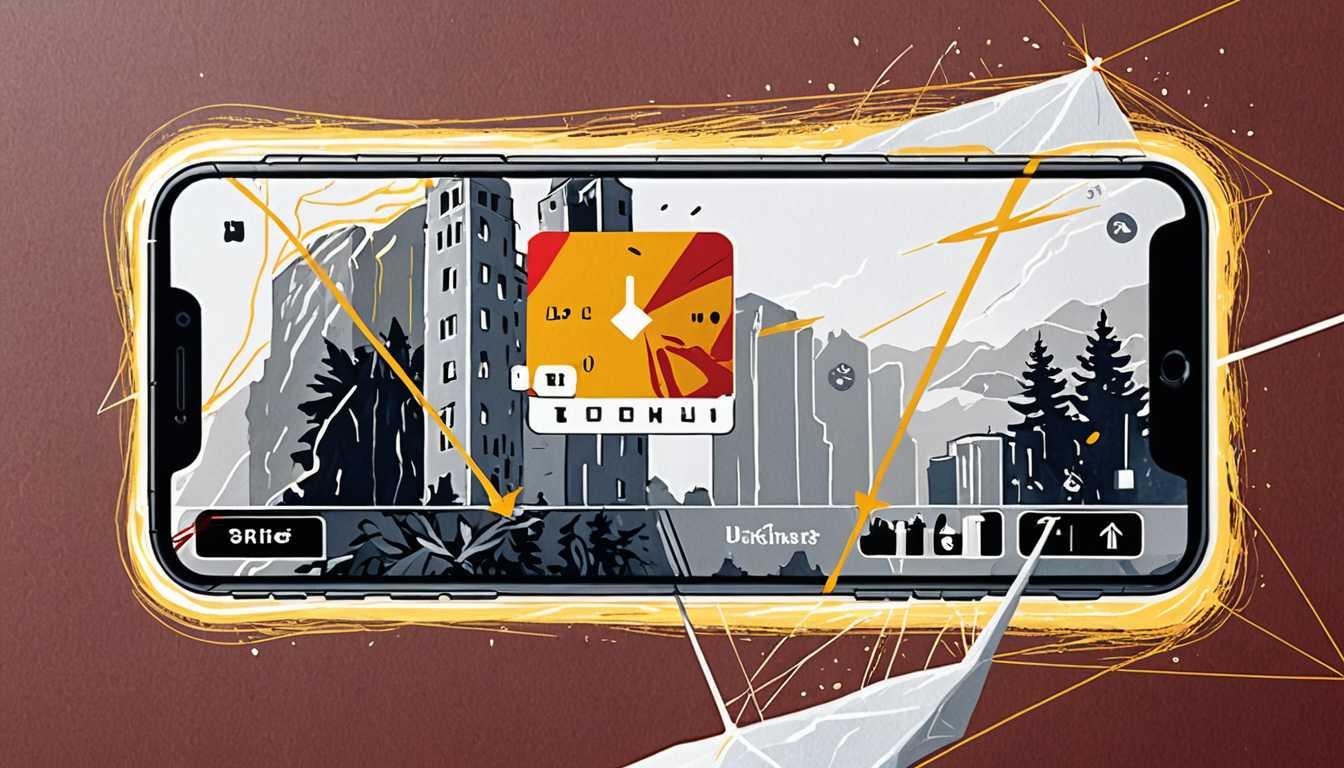
AI Magic: Finding Actions in Videos Fast!
May 2024
MIT News
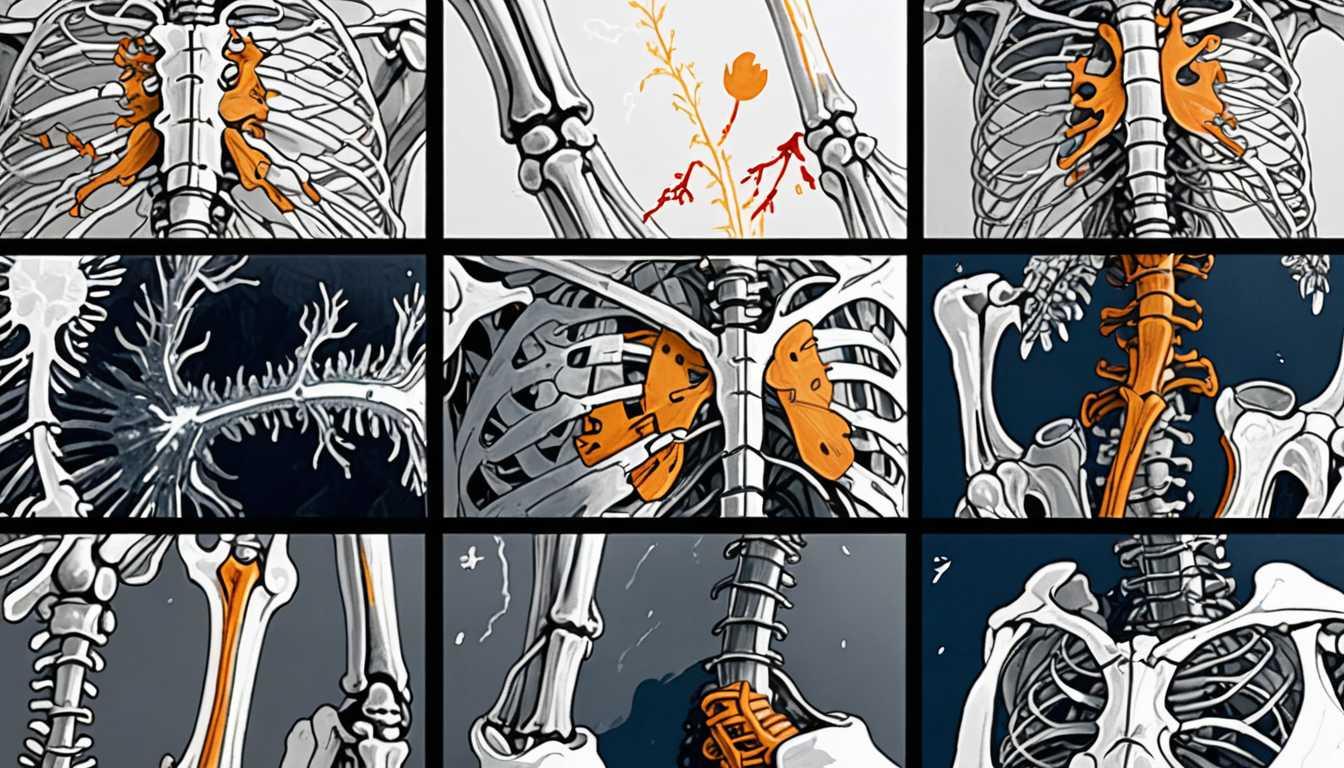
Unveiling Bias in Medical AI Models
July 2024
MIT News
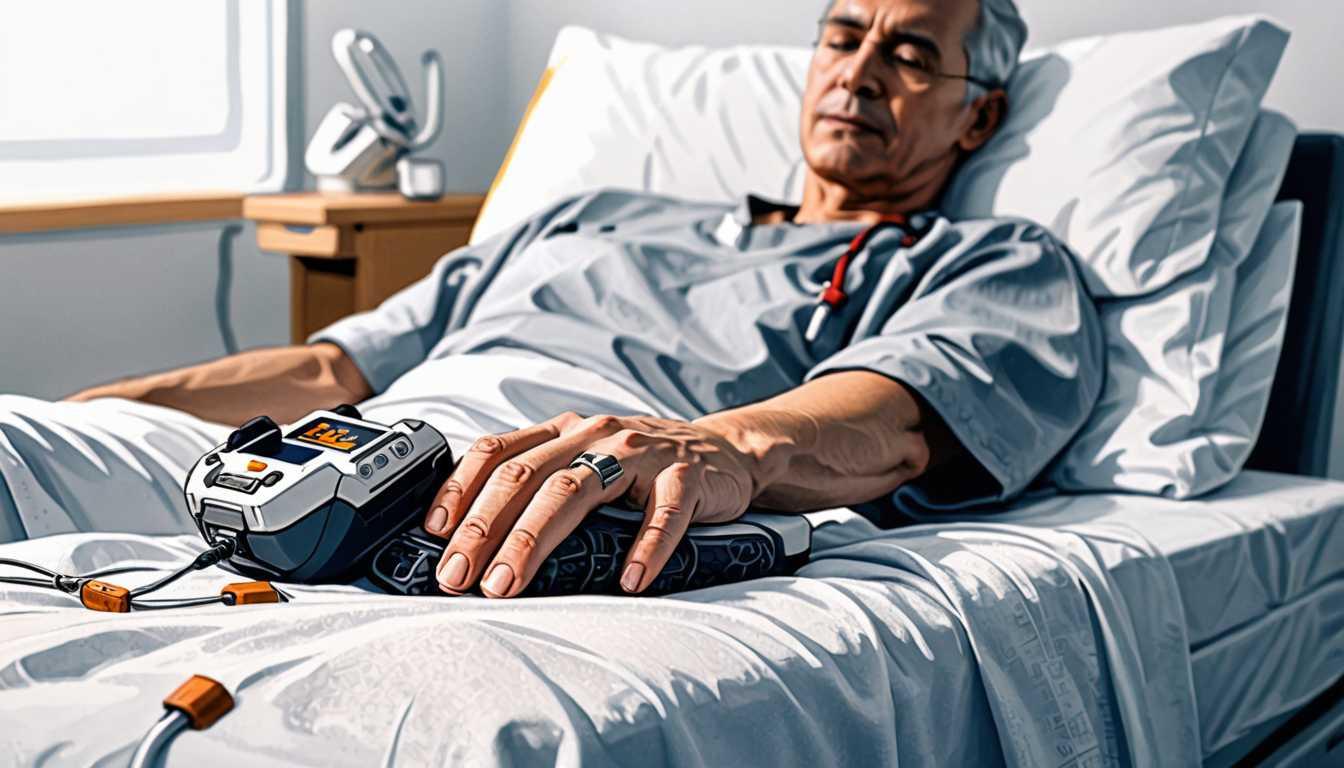
Rethinking Pulse Oximeters: A Fairer Future
February 2024
MIT Technology Review
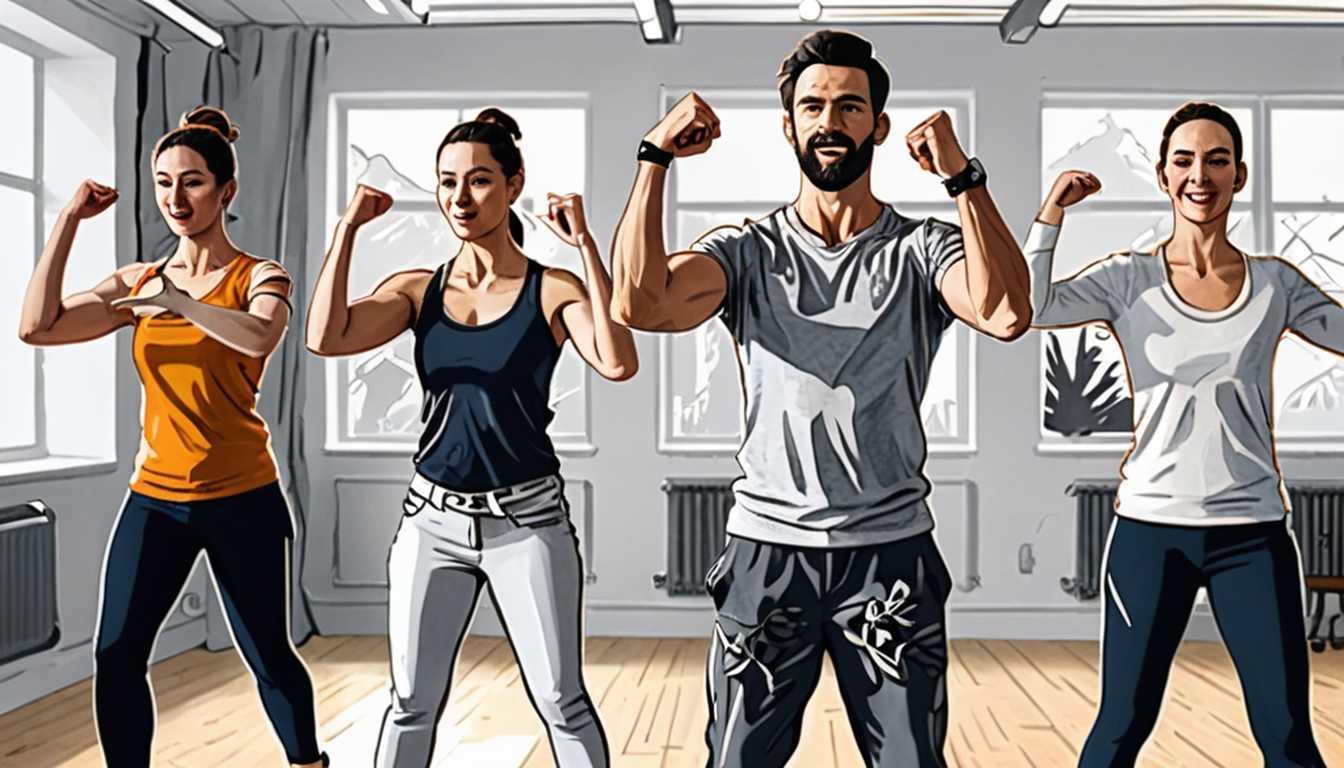
Smart Threads: Clothing That Captures Motion
July 2024
University of Bristol