Beyond Broad Labels: Revealing Health Disparities
August 2023
Cornell University
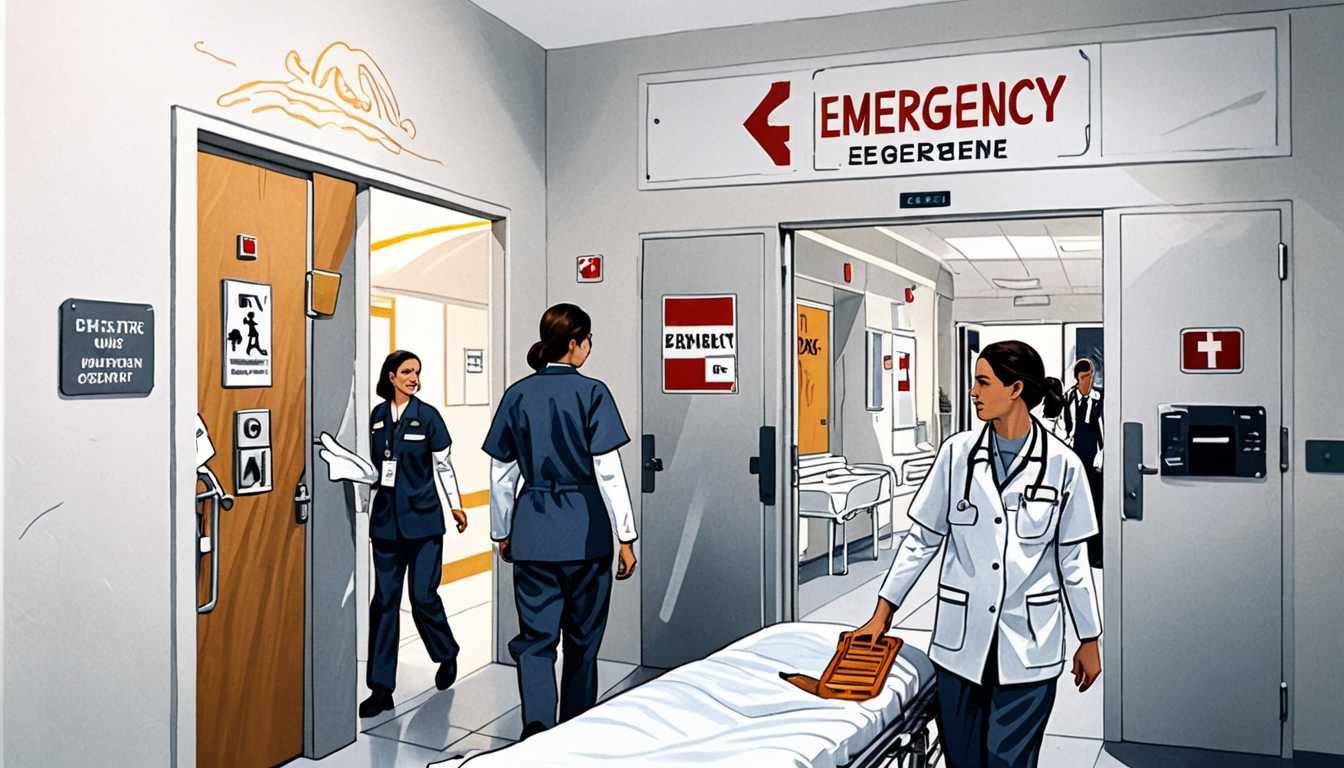
Introduction
Dive into the intriguing world of medical mysteries with Cornell University's latest study, revealing how broad race categories in medicine mask true health risks. Ever wondered why some people get better healthcare than others? This study shows that using general labels like Asian or Black hides the specific health risks faced by subgroups within these categories. With a mix of humor and seriousness, let's uncover the hidden disparities in healthcare and why it's crucial to pay attention to the details. Ready to challenge what you thought you knew about medicine?
READ FULL ARTICLEWhy It Matters
Discover how this topic shapes your world and future
Unveiling Hidden Stories in Health Data
Imagine going to a doctor and being given a treatment based on what works for most people who share your broad racial category, like "Asian" or "Black." But what if your specific background, say "Vietnamese" or "Nigerian," means you have different health risks? This scenario highlights why understanding the nuances within broad race categories in medicine is crucial. It's about ensuring everyone gets the care best suited to them, not just a one-size-fits-all solution. This topic is not just about numbers and categories; it's about real people's lives and how we can make healthcare fairer and more effective for everyone, including you and your community. It's a fascinating mix of science, technology, and social justice, showing how deeply interconnected our world is.
Speak like a Scholar

Clinical Risk Scores
These are calculations used by doctors to understand how likely it is that a patient will have a bad health outcome. It's like a health forecast for patients.

Subgroups
These are more specific categories within a broader group. For example, "Japanese" and "Korean" are subgroups within the "Asian" category.

Disparities
This term refers to differences that aren't fair. In healthcare, it means some groups might get worse care or have poorer health outcomes than others.

Algorithmic Fairness
This is about making sure computer programs make decisions in a way that is fair to everyone, no matter their race, gender, or other characteristics.

Machine Learning
A type of computer science that allows computers to learn from data and make predictions, like forecasting a patient's health risks without being explicitly programmed to do so.

Healthy Immigrant Effect
An observation that immigrants often have better health outcomes than residents of the same ethnicity who were born in the country. It's a curious phenomenon seen in many places around the world.
Independent Research Ideas

Comparing Clinical Risk Scores Across Different Countries
Investigate how clinical risk scores are used in different countries and how cultural and racial diversity is addressed. This could reveal interesting contrasts and similarities in global healthcare practices.

The Role of Social and Environmental Factors in Health Disparities
Explore how where people live, their jobs, and their social conditions affect their health and how this might influence the effectiveness of clinical risk scores. It's a deep dive into the social determinants of health.

Machine Learning and Personalized Medicine
Research how machine learning could be used to create personalized treatment plans based on a person's unique characteristics, including their specific racial or ethnic background. This is at the cutting edge of making medicine more personal and effective.

The Impact of the Healthy Immigrant Effect on Public Health Policies
Study how the healthy immigrant effect influences public health strategies and policies in different countries. It's a fascinating intersection of immigration and health policy.

Ethical Considerations in Algorithmic Fairness in Healthcare
Examine the ethical dilemmas involved in using algorithms in healthcare, especially in ensuring fairness across all racial and ethnic groups. This topic is perfect for those interested in the intersection of ethics, technology, and medicine.
Related Articles
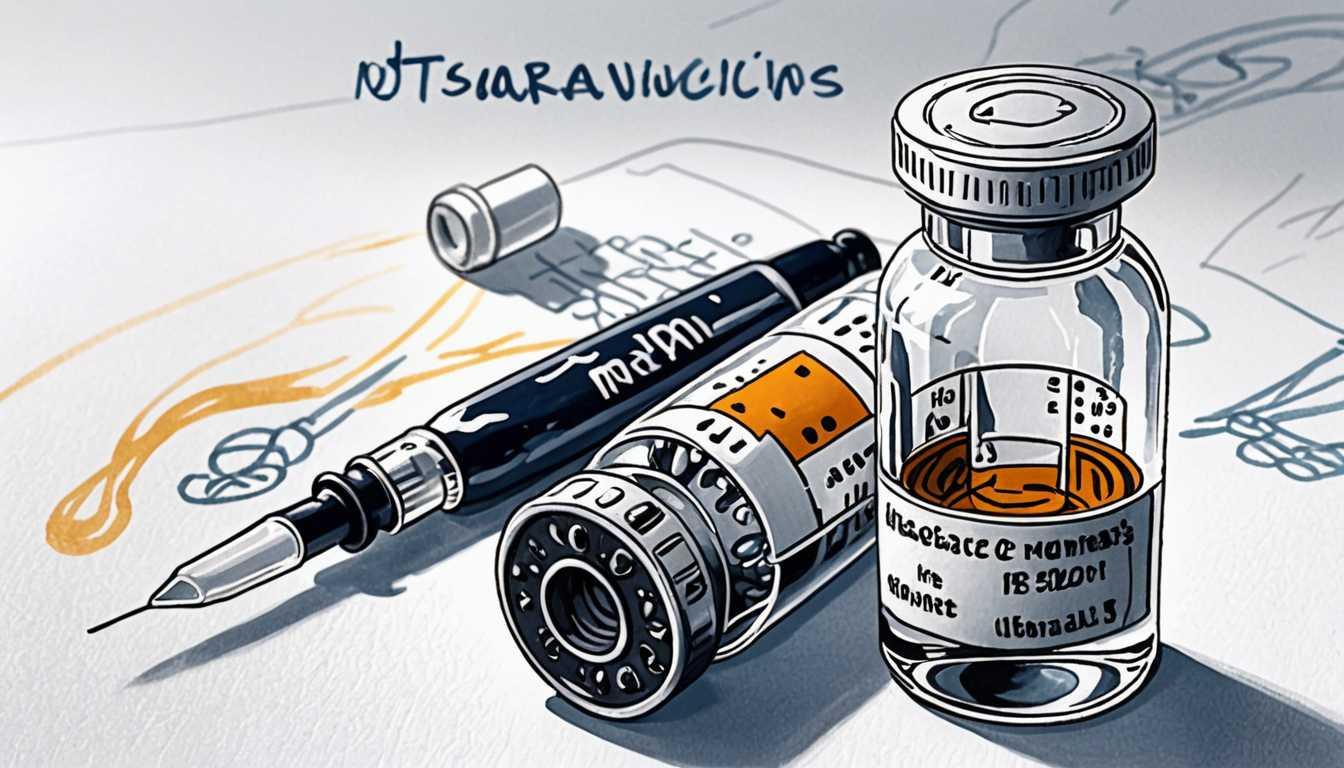
Outsmarting Pandemics: A New Vaccine Play
June 2023
Cornell University
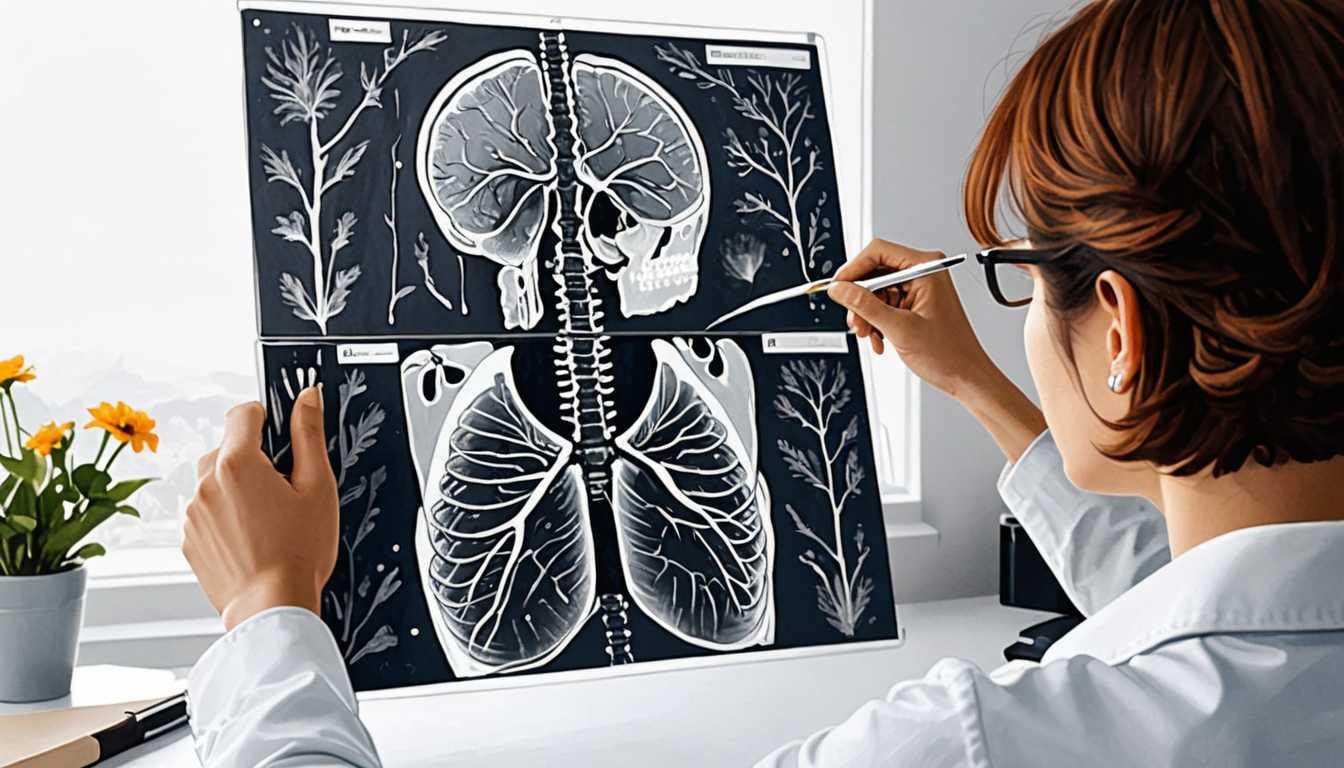
Mia: AI's Triumph in Cancer Detection
November 2023
Imperial College London
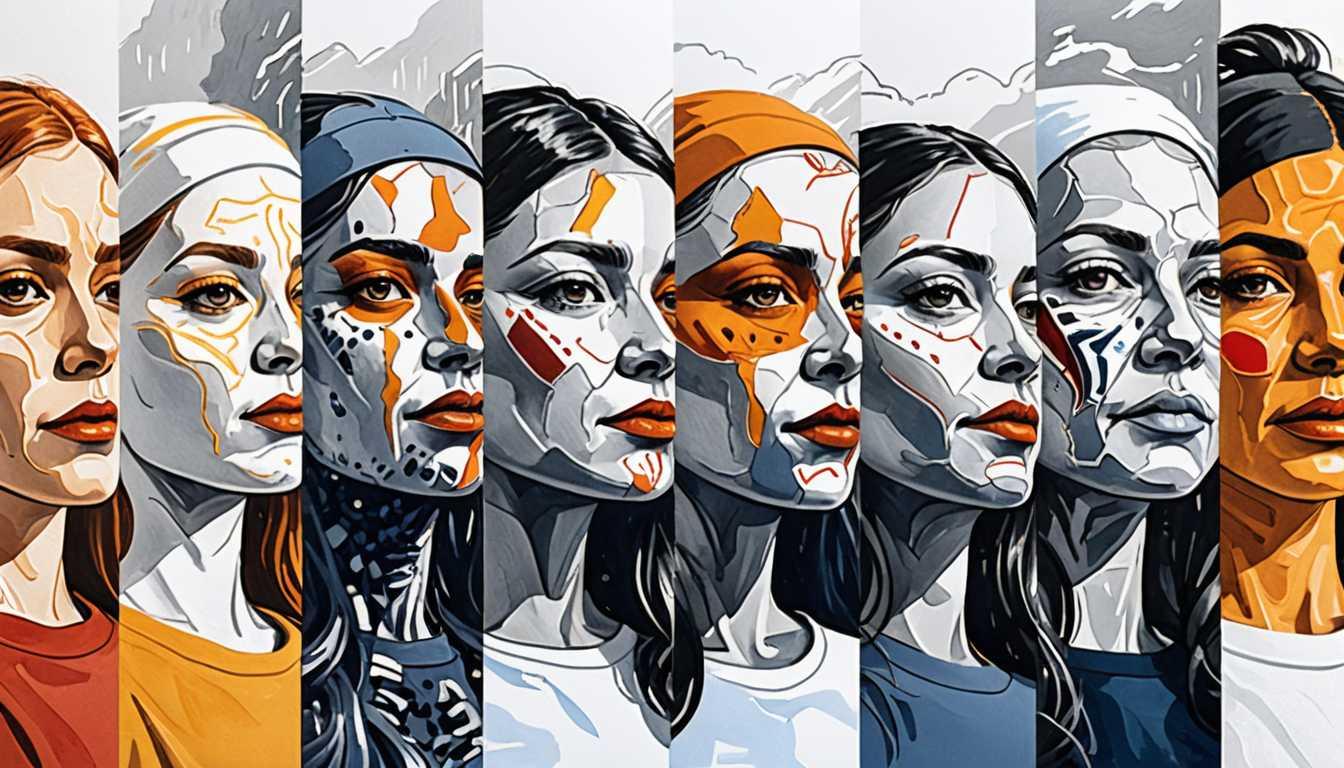
AI Bias in Healthcare Unveiled
August 2023
Massachusetts Institute of Technology (MIT)
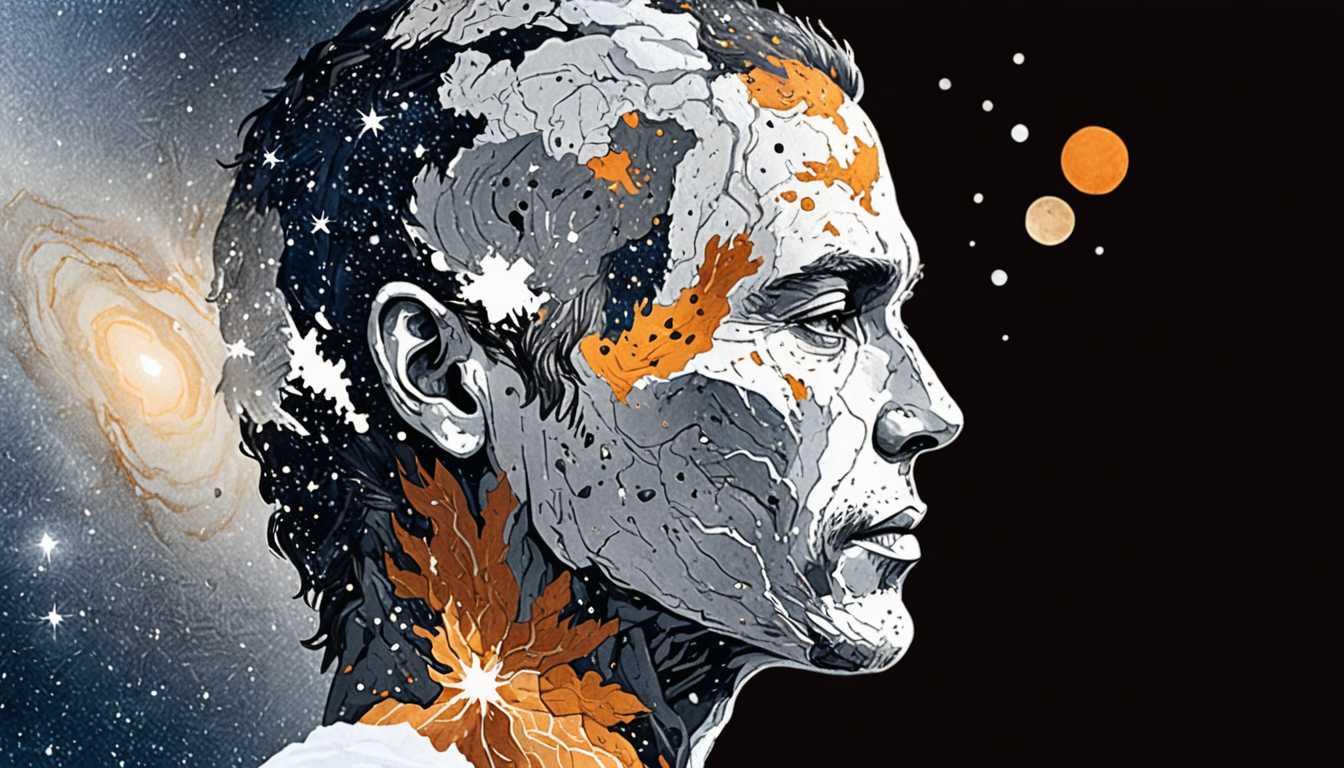
Parkinson's Subtypes Unveiled: Machine Learning Breakthrough
July 2024
Cornell News Highlights
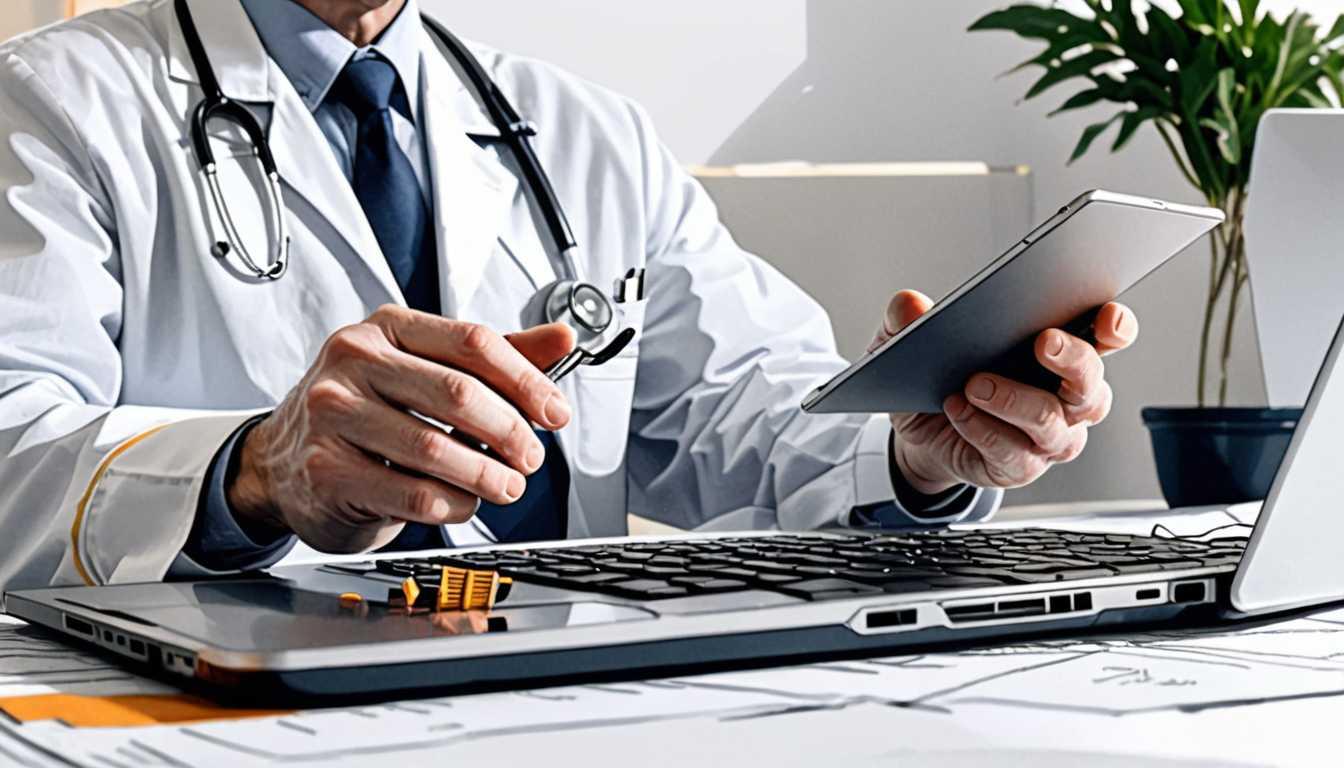
Telemedicine Slashes Hospital Revisits
April 2024
Imperial College London