Resistor Revolution: Rethinking Machine Learning Circuits
June 2024
MIT Technology Review
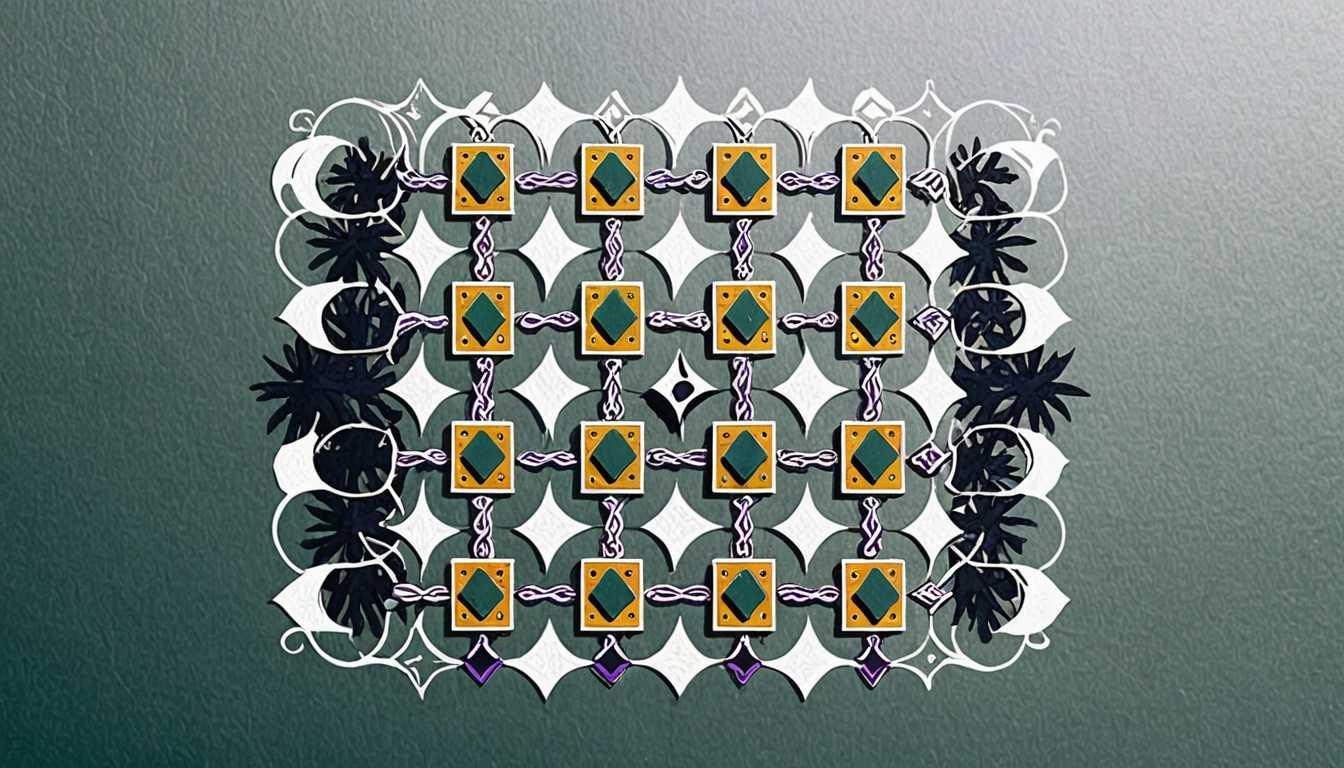
Introduction
Hey there, tech enthusiasts! Dive into this fascinating article from MIT Technology Review, where physicist Sam Dillavou is transforming a bunch of resistors into a potential game-changer for machine learning! Picture a DIY project that could outsmart energy-hungry GPUs while sorting flowers like a pro. With a sprinkle of humor and some clever circuitry, this low-power alternative is on a mission to reshape how we think about computing. Ready to get inspired? Check it out!
READ FULL ARTICLEWhy It Matters
Discover how this topic shapes your world and future
Unleashing the Power of Analog Computing
Have you ever wondered how computers can learn and make decisions? The groundbreaking work of physicist Sam Dillavou at the University of Pennsylvania is paving the way for a new kind of computing. Unlike traditional computers that rely heavily on energy-draining chips, Dillavou's circuit uses simple components called resistors to perform machine learning tasks. This innovative approach could lead to energy-efficient computers that power everything from smartphones to AI systems, helping tackle global challenges like climate change. By understanding how this technology works, you can see how it might impact your life in the future, whether it’s through smarter devices or advancements in fields like healthcare and climate science.
Speak like a Scholar

Machine Learning
A type of artificial intelligence that allows computers to learn from data and improve their performance over time without being explicitly programmed to do so.

Resistor
A component in an electrical circuit that limits the flow of electric current, helping to control the voltage and current levels.

Analog Computer
A type of computer that processes information as a continuous range of values, rather than in discrete steps like traditional digital computers.

Neuromorphic Computing
An approach to computing that mimics the way the human brain operates, using networks of artificial neurons to process information.

Supervised Learning
A method in machine learning where the model learns from labeled data, receiving feedback on its predictions to improve accuracy.

Emergent Learning
A phenomenon where complex behaviors or patterns arise from simple rules followed by individual components, like how a group of resistors can collectively solve complex problems.
Independent Research Ideas

The Future of Energy-Efficient Computing
Investigate how different types of computing can reduce energy consumption and their potential impact on the environment. This topic offers insights into sustainable technology solutions.

Comparing Analog and Digital Systems
Explore the advantages and disadvantages of analog vs. digital computers in various applications, including AI and everyday devices. This could reveal surprising insights into the future of technology.

The Role of Feedback in Learning Systems
Delve into how feedback mechanisms in both machines and humans contribute to learning and adaptation, linking psychology with technology.

Neuromorphic Designs in Robotics
Study how neuromorphic computing can enhance robotic systems, making them more efficient and capable of complex tasks, which could lead to innovations in automation.

Emergent Behavior in Complex Systems
Research how simple rules can lead to complex outcomes in both electronic circuits and biological systems, fostering a deeper understanding of systems thinking in science.
Related Articles
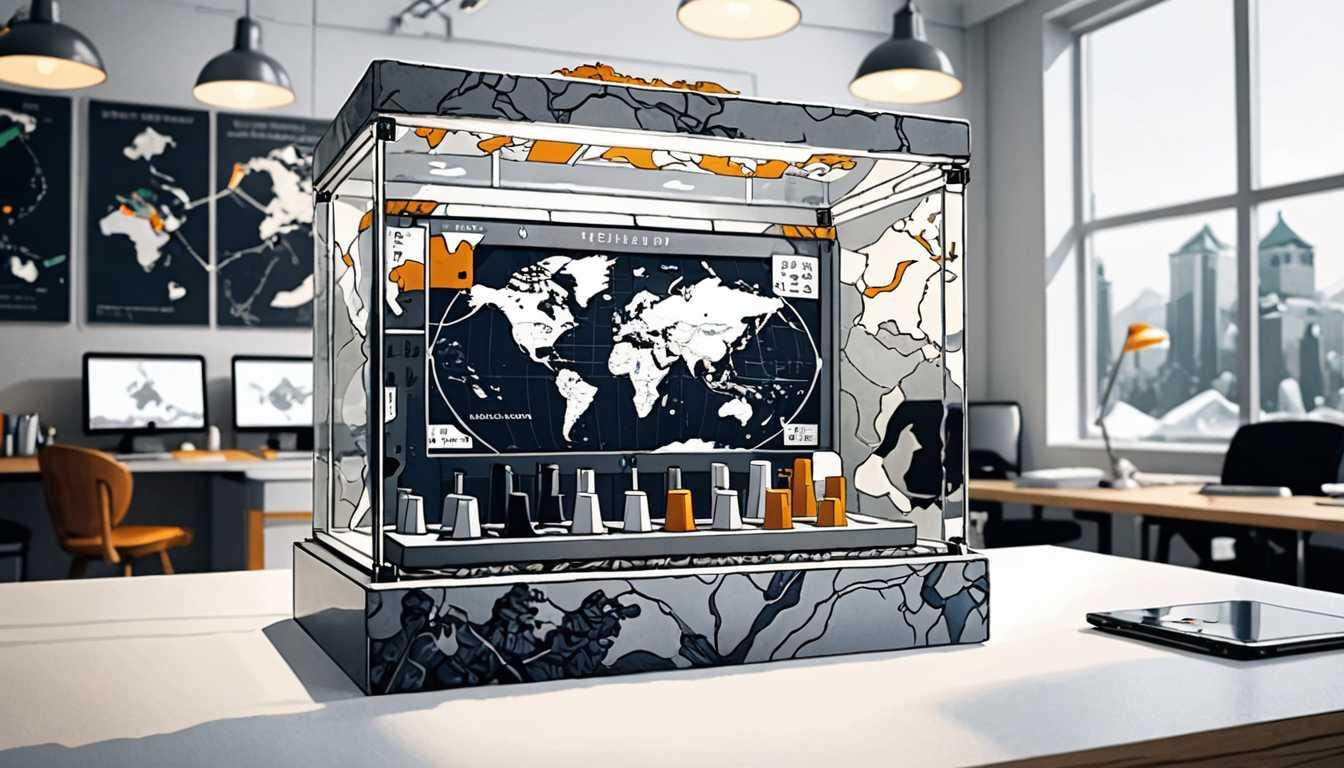
Forecasting the Future: AI Meets Weather Science
July 2024
MIT Technology Review
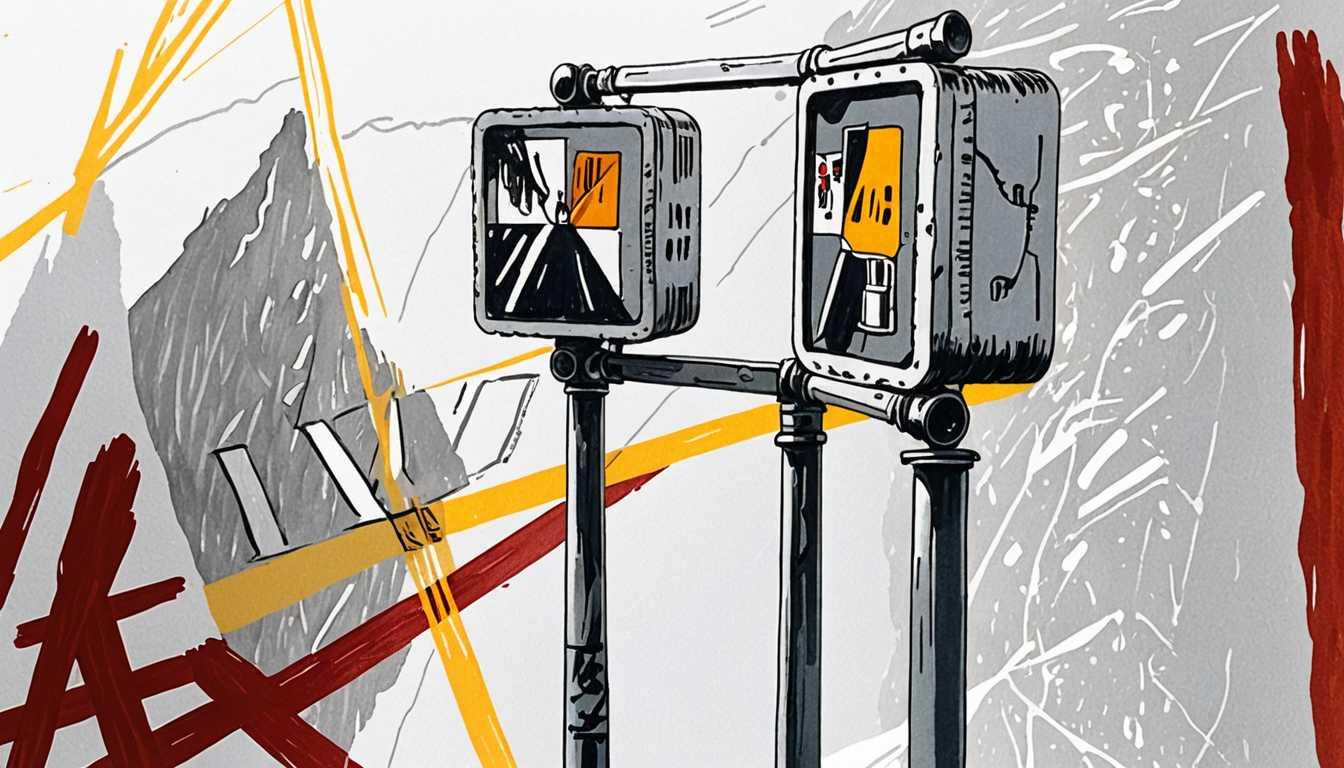
Beyond Captchas: Proving Humanity
October 2023
MIT Technology Review
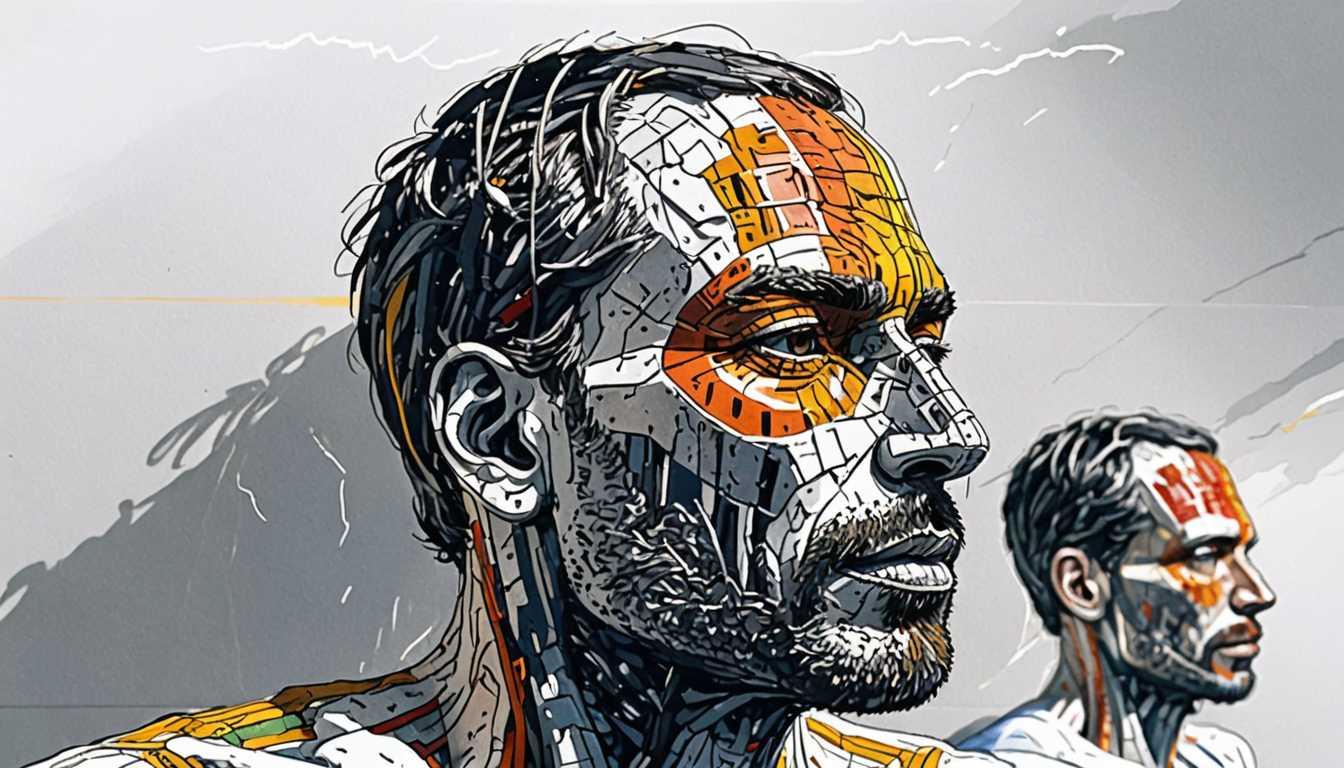
From Blurry to Brilliant: MIT's Discovery
January 2023
Massachusetts Institute of Technology (MIT)
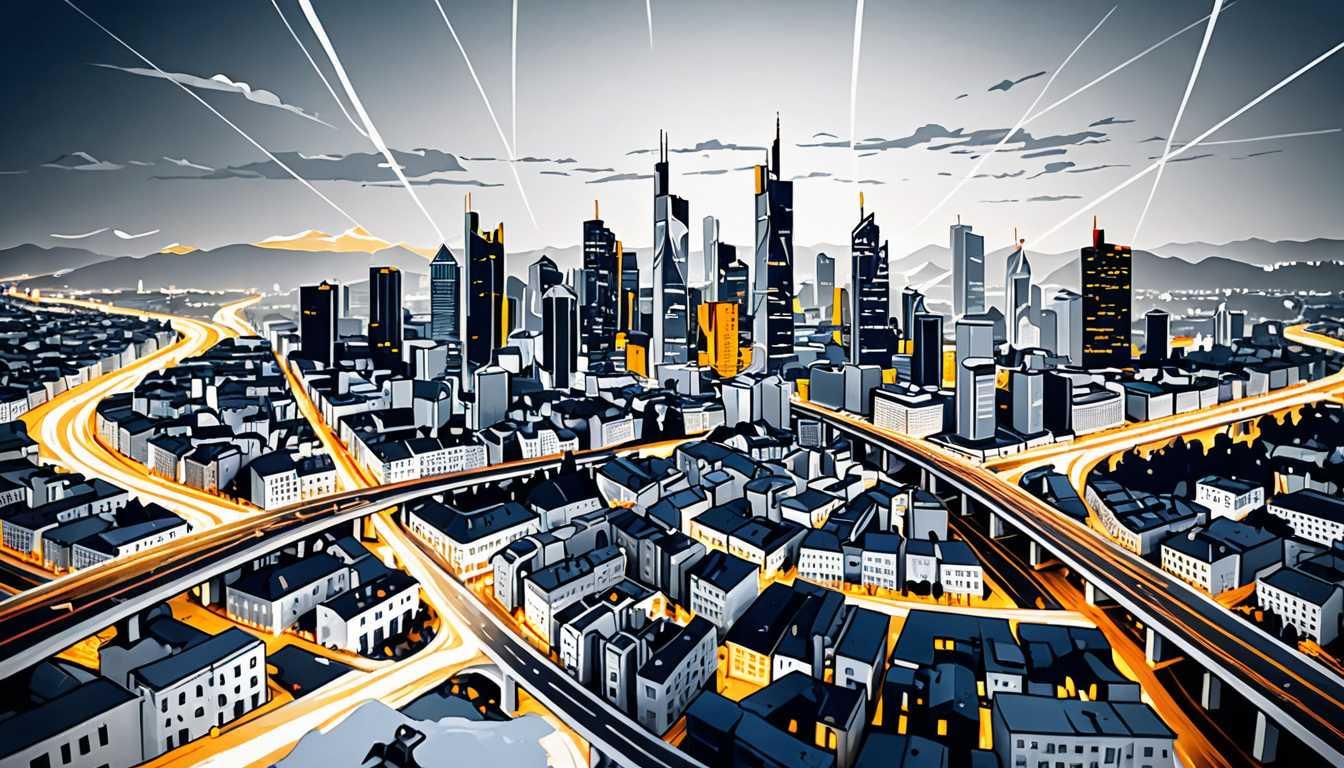
Unlocking the Future of the Internet
May 2024
Harvard Gazette
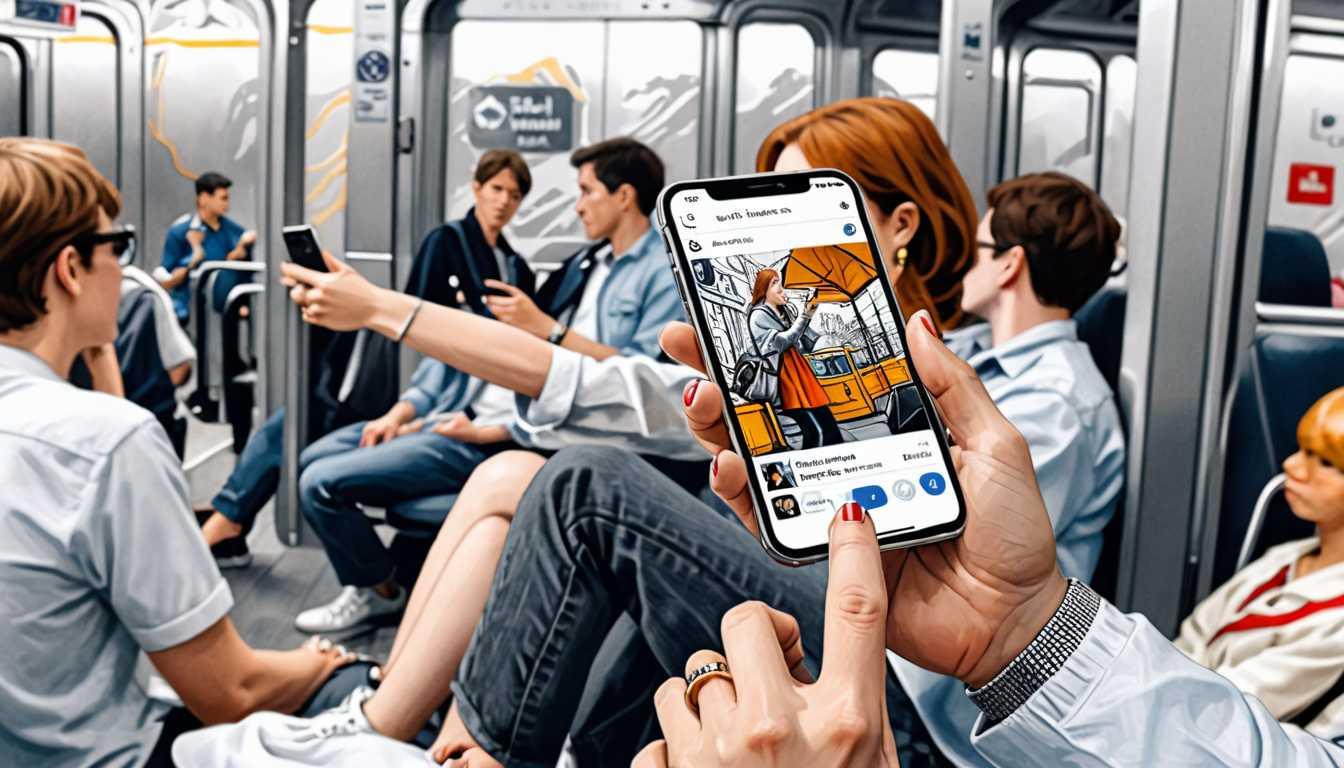
Master Your Social Media Feed
May 2023
The Conversation