AI Reasoning: Beyond Memorization
July 2024
MIT News
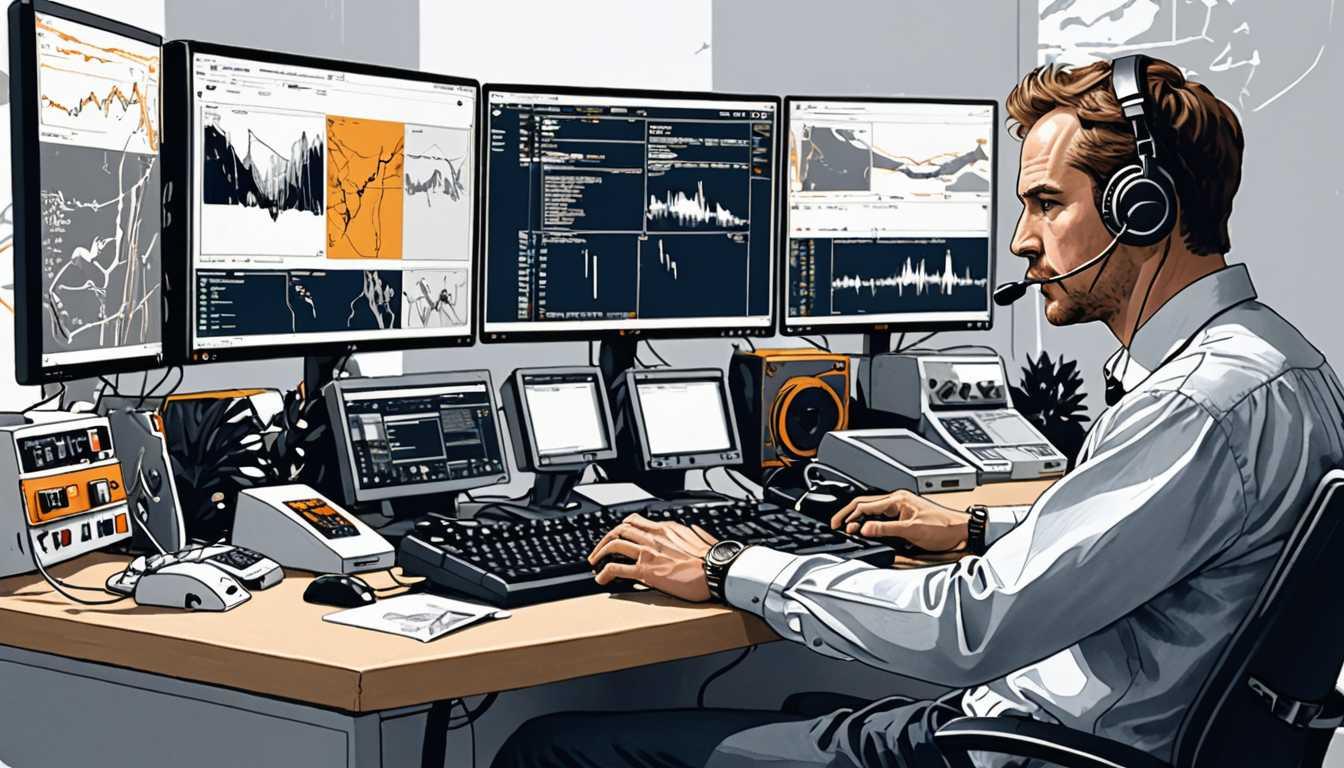
Introduction
Ever wondered if those smart AI language models truly understand or just memorize? MIT researchers dug deep into the minds of large language models (LLMs). They found out these models are like experts in familiar scenarios but struggle in new ones. Dive into the mystery of AI reasoning skills in this mind-boggling article by Rachel Gordon from MIT CSAIL.
READ FULL ARTICLEWhy It Matters
Discover how this topic shapes your world and future
Navigating the Minds of Language Models
Artificial Intelligence is a fascinating field that delves into the capabilities of machines to understand and process human language – a skill that has far-reaching implications in various aspects of our lives. In a recent study by MIT researchers, the focus was on Large Language Models (LLMs) like GPT-4 and Claude, which have garnered attention for their impressive performance in familiar tasks but struggle when faced with novel scenarios. This study sheds light on the intricate balance between memorization and reasoning skills in these models, challenging the assumptions about their true reasoning abilities. Understanding the limitations of these models is crucial as we aim to enhance their adaptability and broaden their potential applications in diverse real-world situations. As AI becomes increasingly prevalent in our society, the need for models that can handle both familiar and unfamiliar scenarios becomes paramount. This research not only highlights the complexities of LLMs but also paves the way for future advancements in AI technology.
Speak like a Scholar

Large Language Models (LLMs)
These are sophisticated AI systems designed to process and generate human language, such as GPT•4 and Claude.

Memorization
The act of storing and recalling information from memory without necessarily understanding or reasoning about it.

Reasoning Skills
The ability to make sense of information, draw conclusions, and solve problems through logical thinking.

Counterfactual Scenarios
Hypothetical situations that deviate from the norm or default conditions, challenging the model's adaptability.

Overfitting
When a model performs well on specific tasks due to memorization of training data, but struggles with generalizing to new or unseen situations.

Interpretability
The degree to which the decision•making processes of AI models can be understood and explained by humans.
Independent Research Ideas

Investigating the Impact of Training Data Complexity on LLMs' Generalization Abilities
Explore how the diversity and intricacy of training data influence LLMs' performance in novel scenarios.

Analyzing the Role of Transfer Learning in Improving LLMs' Adaptability
Examine how pre•existing knowledge can be effectively transferred to enhance LLMs' capabilities in unfamiliar tasks.

Exploring the Ethical Implications of LLMs' Limited Generalization
Delve into the ethical considerations surrounding the potential biases and limitations of LLMs in real•world applications.

Investigating the Development of Explainable AI Models for Improved Interpretability
Explore methods to make AI decision•making processes more transparent and understandable to users.

Examining the Impact of Task Complexity on LLMs' Performance
Investigate how the complexity of tasks influences LLMs' ability to reason and generalize across different scenarios.
Related Articles
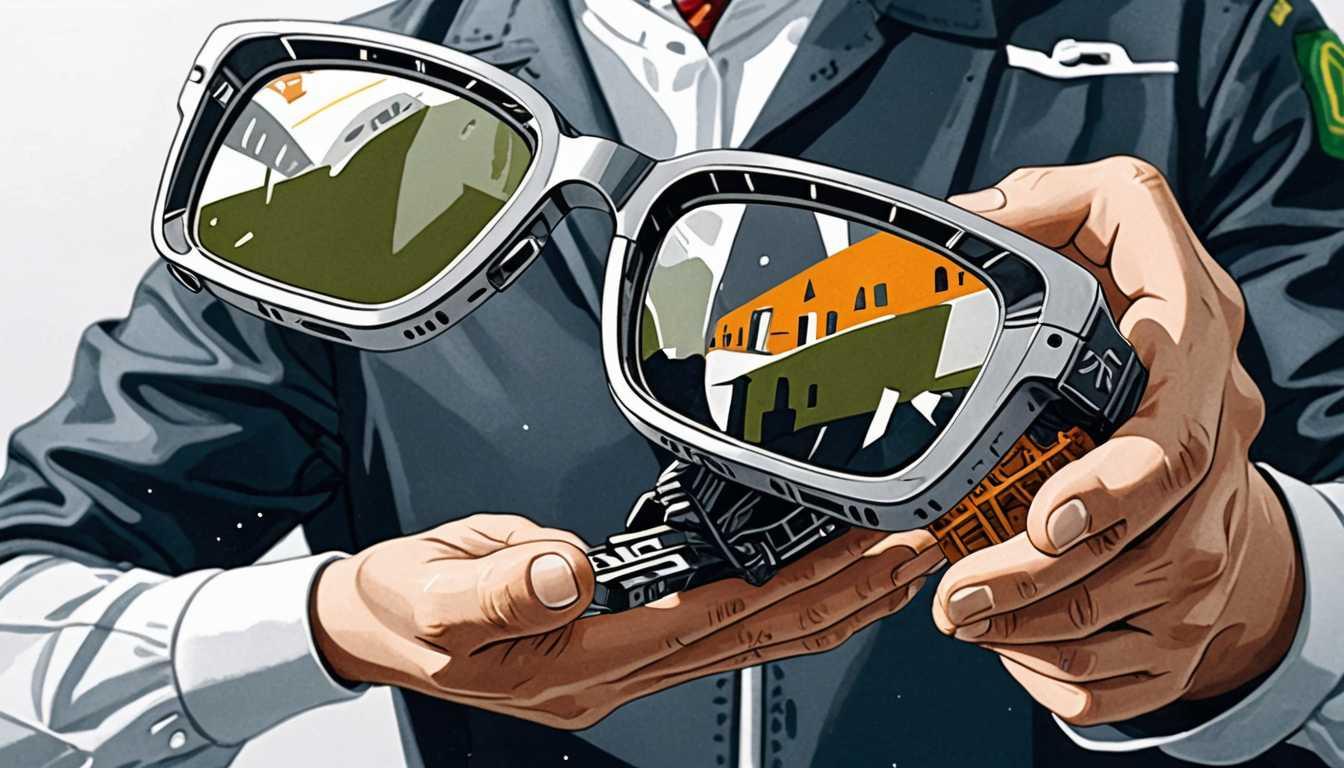
Silent Commands: Future Eyewear Unveiled
April 2023
Cornell University
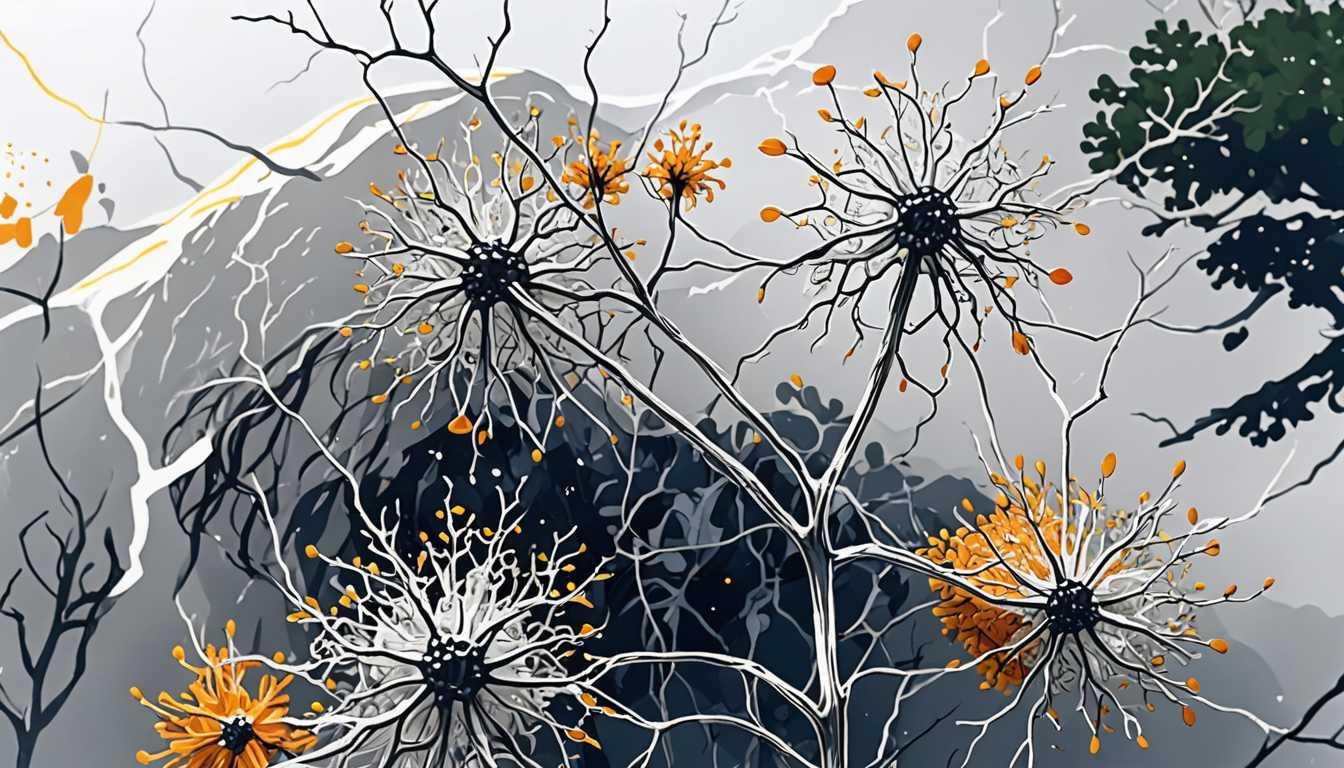
AI Learns to Sidestep Toxicity
April 2024
Massachusetts Institute of Technology (MIT)
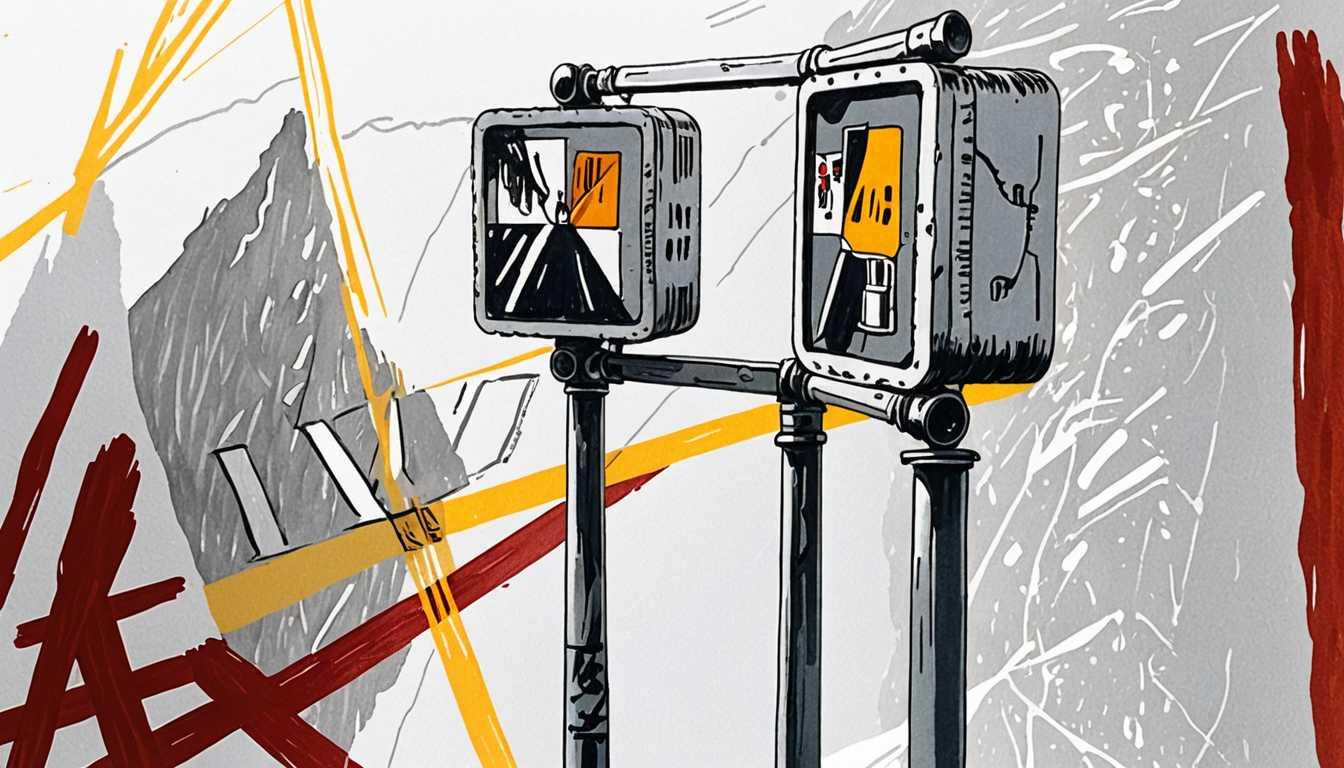
Beyond Captchas: Proving Humanity
October 2023
MIT Technology Review
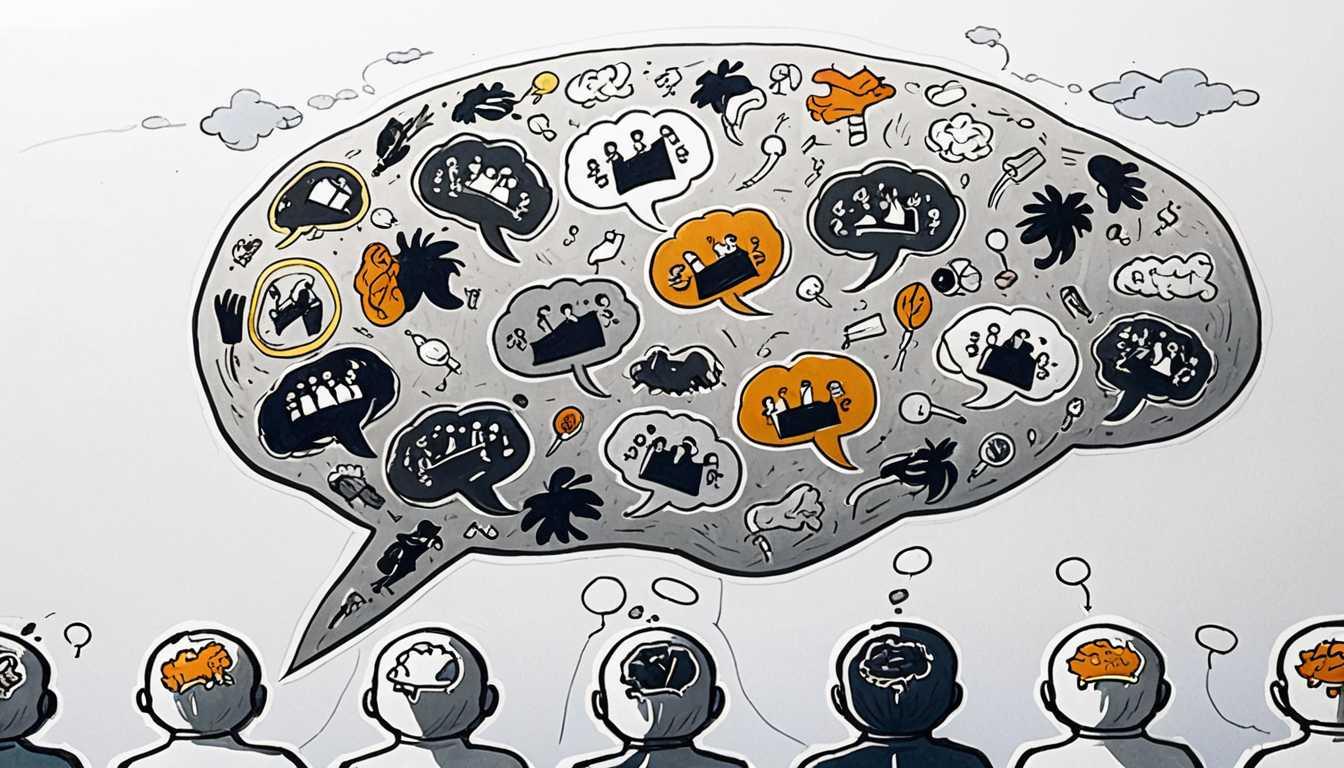
Logic: The AI Bias Buster
March 2023
Massachusetts Institute of Technology (MIT)
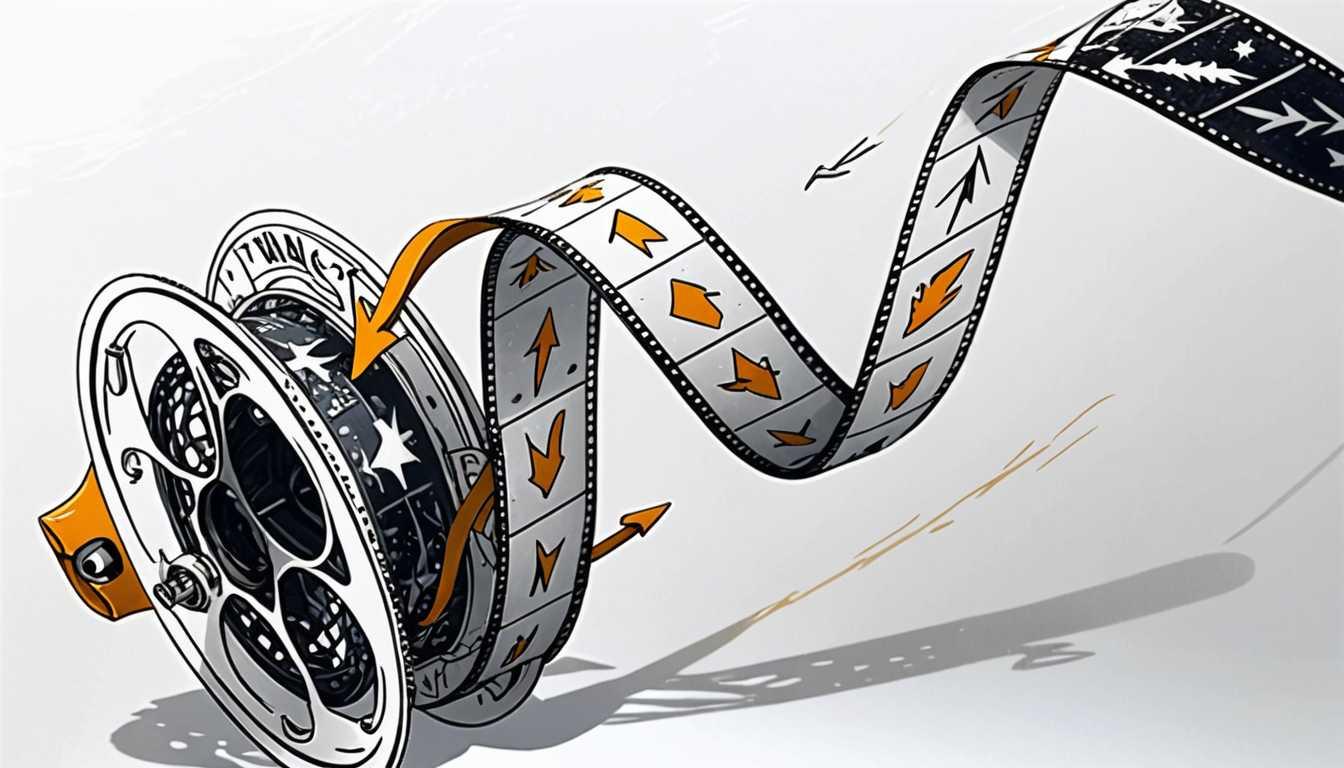
Magic Wand for Videos: DynIBar Revealed
July 2023
Cornell University